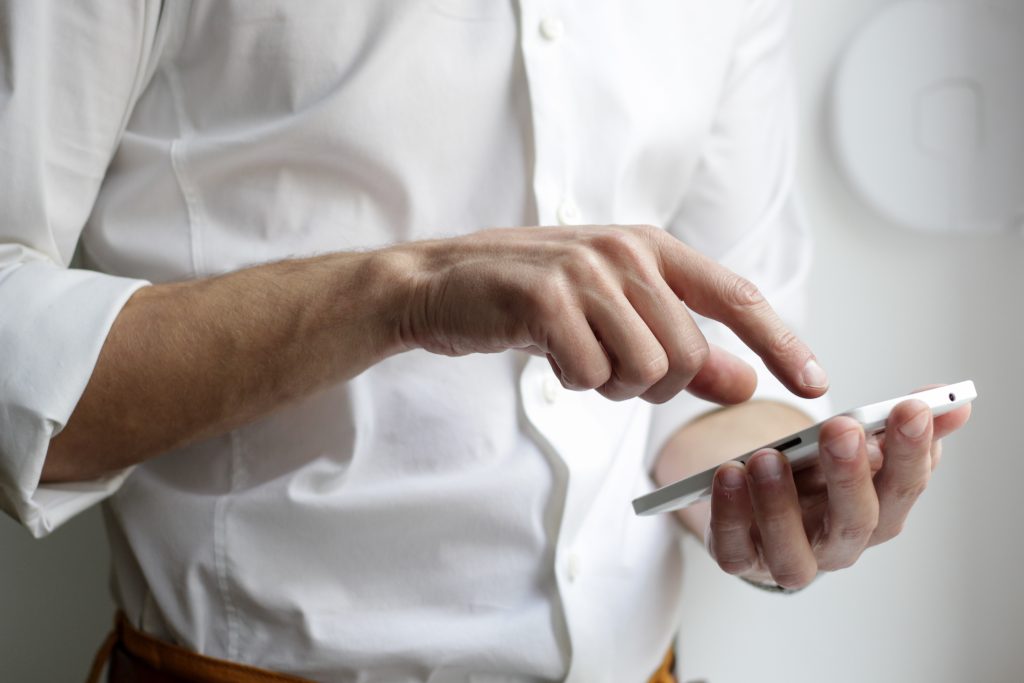
(Person holding white android smartphone in a white shirt, Norwood themes, CC by attribution)
Data-driven platform businesses (DDPBs) are also referred to as data-driven business models (DDBMs) that amplify the incorporation of data processing techniques and diverse digital enablers (Hilbig, Etsiwah, Hecht, 2018, p. 3). Such as employing artificial intelligence (AI), automations, IoT, cloud computing, and big data (Hilbig, Etsiwah, Hecht, 2018, p. 3).These methods can have significant impact on the way companies create, deliver, communicate, and capture value centred around innovative value propositions (Hilbig, Etsiwah, Hecht, 2018, p. 3). Ultimately, it acts as a major factor contributing to wider processes known as datafication and digitalisation (Mansell, Steinmueller, 2020, p. 35).The multisided market consists of the two ways to understand the structure of different customer groups which can be done through including all customer groups in a single market or defining a separate market for each group (Gürkaynak, Inanilir, Diniz, Yasar, 2016, p. 103). The multisided market comprises four major elements; the platforms, the users that are gathered together through the platforms, the platforms pricing structure, and the platforms aim to benefit from the effects on the network (Gürkaynak, Inanilir, Diniz, Yasar, 2016, p. 105).The operations of DDPBs such as Facebook effectively, convert the traditional processes of the online environment and build new relationships and methods (Mansell, Steinmueller, 2020, p. 35). Through leveraging multisided market models (MMM), factors such as their market dominance, influence on industries/society, and their data-driven business models ultimately, establish the economic and societal implications of DDPBs.
The DDPBs, Facebook leverages multisided market models by combining multiple distinct user groups in order to generate value for each other. The social media platform, Facebook offers free services to users and generates a revenue by relying on concepts of multisided markets that emphasises the combining of multiple user groups. Concepts such as, the two-sided market model (TSMM) and network effects (Mansell, Steinmueller, 2020, p.38). Facebook act as a device that simplifies transactions between users and advertisers this improves the ‘liquidity’ of the market by enhancing the transactions of profitable volume between buyers and sellers thus, contributing to creating a larger and more stable market (Thépot, 2013, p. 202).In neoclassical economics TSMM is perceived as two markets on a platform; one for users to post content and the other offers a channel for advertisers aiming to reach and message customers (Mansell, Steinmueller, 2020, p.38). Facebooks revenue derives from advertising as they primarily focus on advertisements and constructing an audience (Thépot, 2013, p. 196). The platform implements TSMM by connecting its users and advertisers for instance, advertisers purchasing advertising space on Facebook have the ability to select their targeted audience. Data available such as location or age contributes to the platforms functionality and assists the increased possibilities of corresponding advertisers and potential buyers interest mutually and profitably (Thépot, 2013, p. 203).Specifically, the option to advertise through ‘sponsored stories’ brings together two user groups as they are content shared by users who interact with a Facebook business page. The content of the story may include the user participating in activities related to the business as well as the brands image and link to their business page, categorising it as a two-sided transaction platform. These images constitutes the advertisement that the business pays for, and the post is presented on the users page to all their contacts. In relation to the advertisers perspective this practice is an effective way of leveraging the network of its customers as the sponsored stories also include recommendations from the users who share them. Similar to search-based advertising, the greater collective of users and advertisers increases the possibilities of effective transactions (Thépot, 2013, p. 203). Despite the advertisements being relevant to users’ profiles they still may be bothered by the sponsored stories feature however, they can alter the consumers experience. As it is categorised as user content shared by the user to their contacts, the advertisement takes on the façade of a non-sponsored post. This value alludes to the network effect, a MMM that outlines how a greater user base and increase of content production enhances the overall system (Thépot, 2013, p.204); Facebook employs this to retain and attract different sides of the market. Reports confirm that 43% of online shoppers claim to have made a purchase due to social network surfing thus, indicating advertises power to reach an active and willing audience (Klaassen, 2009, p. 2).
As initially discussed, Facebook leverages various MMMs simultaneously, whilst demonstrating the models’ societal implications for instance, data security and privacy issues illustrates user concerns of tracking and data collection. Facebook acting as a three-sided platform consists of the company selling users data to third parties. Access to this data depends on users’ willingness to share it which the platform lacks control over however, it does have partial persuasion over consumers reactions to their data being sold (Filistrucchi, 2018, p. 7). Failing to inform users of this can increase Facebooks profits as users would not perceive the negative impacts and continue to provide consequently, not providing these insights is a consumer protection issue and affects the platforms pricing strategies (Filistrucchi, 2018, p. 7). This becomes relevant when considering the implementation of consumer protection or competition policy. These issues stimulate notions and arguments surrounding content moderation and free expression specifically, the challenges to balance data protection and content moderation. As data collection is used to enforce community standards for content moderation yet, extensive data collection can appear as invasive affecting users’ privacy (Filistrucchi, 2018, p. 10). Criticism and debate around Facebooks contribution to spreading misinformation and fake news gained a significant amount of attention during the 2016 US presidential election. In particular, a fake news story had been widely spread claiming that the Pope Francis had endorsed Donald Trump for president.
Click to view Facebook post…
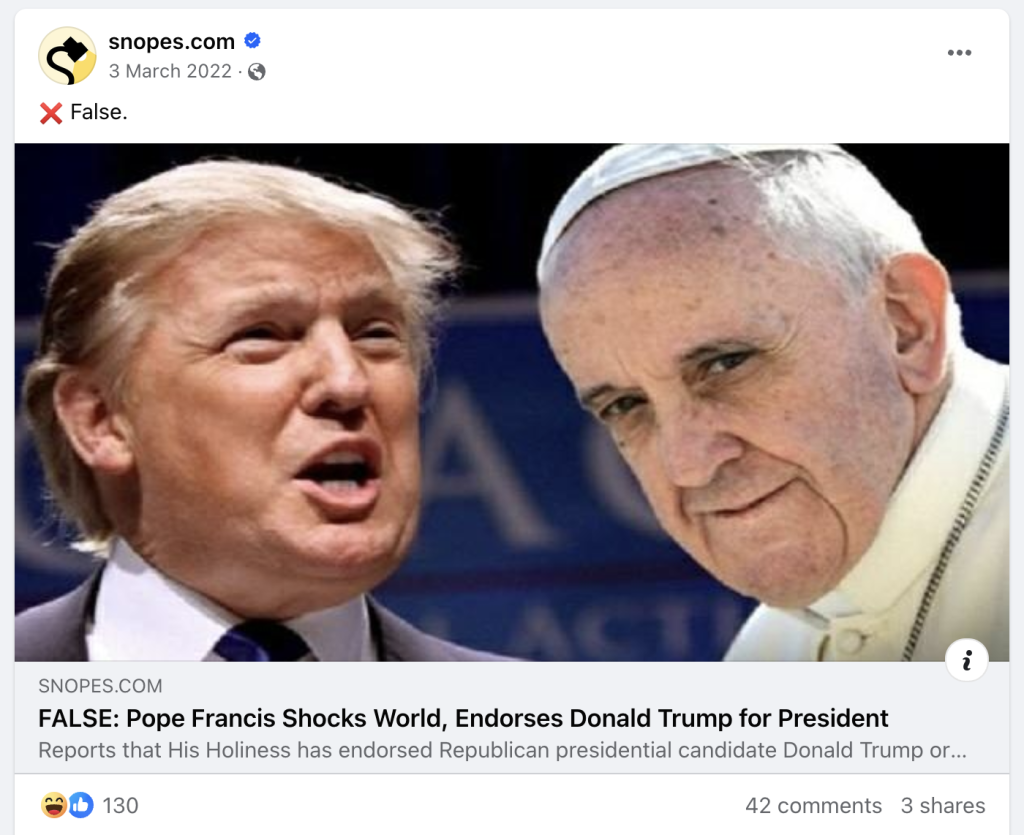
This incident aligns with principles supporting freedom of expression as political actors communicate their messages even though it is untrue or controversial. On the contrary, this entirely fabricated accusation unveils fears of the platforms content moderation responsibilities to prevent distribution of false information. This example of fake news also touches on the impacts of echo chambers and filter bubbles on information consumption and polarisation as well as issues surrounding algorithmic biasness and consequences on inclusion and diversity. Online platforms and algorithms construct filter bubbles by basing users feed on their previous online behaviours. Interacting with the post mentioned above will contribute to personalising users’ content and expose them to more fake news as well as echo chambers; an online environment that allows them to interact with other users who share similar ideas. These concepts will reinforce and promote misleading information and cause isolation of information that could challenge views as well as users restricted exposure to diverse opinions. Repetitive ideas are intensified by algorithmic biasness which increases polarisation and reflects the lack of inclusion and diversity. Ultimately, these societal implications related to the three-sided platform model indicates the insights to purchasing data due to its ability to expose the public to misinformed content that signifies the malleability of their voting perceptions.
Facebooks use of the TSMM reflects economic implications and is a prominent example of surveillance capitalism (SC), [a political economic concept that examines the prevalent system of commodification and collection of personal data by corporations to gain power and wealth whilst, portraying a major threat to democratic principles and individual rights (Zuboff, 2018, p. 8)]. SC subtlety migrates from the online world into our everyday lives due to the competition to manufacture products that accurately predict human behaviour. The gathering of behaviour data (raw material) is an aggressive act referred to as “personalisation” which becomes insufficient due competition intensifying (Zuboff, 2018, p. 27). SC incorporates automated systems to influence and modify human behaviour in hopes to favour their commercial outcomes which Facebook mirrors through its highly controversial “contagion experiment” in 2012. Its ethical concerns emulate surveillance profits impact to disregard individuals autonomy and democratic order thus, challenging online and real-life traditional processes. SC interrelates with network effects as the volume of data collected is co-dependent on the quantity of users hence, producing a dominant platform through surveillance capabilities and personalisation.
Advertisers challenge traditional advertising through nano-targeting a market in a unique way (Roberts, 2010, p. 26). Micros-targeting methods effectiveness is demonstrated in an experiment that Lessin conducted in 2008. The media industries profits and engagement rely on Facebook and algorithms to maintain their content as relevant and to reach consumers therefore, they have had to adapt their practices to conform within the digital age. Tech giants remain dominant control over users data which initiates antitrust and competition concerns for instance, Facebooks ownership of WhatsApp and Instagram. This illustrated a lack of competition that provided them with more supremacy in the digital advertising market. This provoked government scrutiny and the enforcement of strategies; harsher policies to protect customer confidentiality and encouragement of competition to create market fairness. Economic benefits of Facebooks network effects provide an increase in personalised advertising options and interactivity for example, businesses possessing easy access to relevant data provides product development and market strategies which increases market efficiency. Overall, Facebooks economic success is a result of its application of the TSMM that signifies the construction of economic value by facilitating relations between various user groups and leveraging its generated data.
DDPBs (Facebook) gain success through leveraging MMMs and provide a critical outlook on the models societal and economic implications ultimately, this reflects its ability to majorly impact the online environment and construct contemporary methods and relationships. This is conveyed through acknowledging their business strategies, consequences of their practice, revenue models, and competition. Assessing data’s role within platform economics, platforms regulation challenges, and the concerns/results for users and the entirety of society assists the analysis of these DDPBs.
References:
- Anchin, M. (2009, September 22). The Nielsen Company and Facebook form strategic alliance [News Release]. Nielsen. http://en-us.nielsen.com/main/news/news_releases/2009/september/the_nielsen_company
- Edwards, J. (2013, June 7). Facebook has finally killed “sponsored stories,” the hated ad format that made it look like you advertised something when you didn’t. Business Insider. https://www.businessinsider.com/facebook-kills-sponsored-stories-2013-6
- Evon, D. (2016a, July 10). Did pope francis shock world by endorsing Donald Trump for president?. Snopes. https://www.snopes.com/fact-check/pope-francis-donald-trump-endorsement/?fbclid=IwAR20aWqQxam1Zuy1tFHBX-cs5sdDtmFPdQEo6wKf4kask8GKE9CxKvXItPo
- Evon, D. (2016b, July 10). Snopes.com – ❌ false. Facebook. https://www.facebook.com/snopes/posts/pfbid032LZFZdwMdePbmvFBUAuKxmGo6cDrqLWZAMwWwbH46qG7SaqN9Rgm5J7LMA7x1PyXl
- Filistrucchi, L. (2018). Hearing on Re-thinking the use of traditional antitrust enforcement tools in multi-sided markets. Market Definition in Multi-Sided Markets , 2–16.
- Gürkaynak, G., İnanılır, Ö., Diniz, S., & Yaşar, A. G. (2016). Multisided markets and the challenge of incorporating multisided considerations into competition law analysis. Journal of Antitrust Enforcement, 100–109. https://doi.org/10.1093/jaenfo/jnw007
- Hilbig, R., Hecht, S., & Etsiwah, B. (2018). The rise of data-driven business models in the Berlin start-up scene. Open Innovation: Bridging Theory and Practice, 353–377. https://doi.org/10.1142/9789811219238_0013
- Learmouth, M., & Klaassen, A. (2009). Facebook’s plan to amp up ad revenue. Advertising Age, 80(4).
- Lessin, S. (2008). Welcome to the world of nanotargeted ads. Target Markets; Social Networks; Online Advertising, 79(42), 1–13.
- Mansell, R., & Steinmueller, W. E. (2020). Economic Analysis of Platforms. In Advanced introduction to platform economics (01 ed., pp. 35–41). essay, Edward Elgar Publishing.
- Meyer, R. (2014, June 28). Everything we know about Facebook’s secret mood-manipulation experiment. The Atlantic. https://www.theatlantic.com/technology/archive/2014/06/everything-we-know-about-facebooks-secret-mood-manipulation-experiment/373648/
- MinuteVideos. (2016). Filter Bubbles and Echo Chambers. YouTube. Retrieved October 17, 2023, from https://www.youtube.com/watch?v=Zk1o2BpC79g.
- Roberts, K. K. (2010). Privacy and Perceptions: How Facebook Advertising Affects its Users. The Elon Journal of Undergraduate Research in Communications, 1, 24–26.
- Selinger, E., & Hartzog, W. (2015). Facebook’s emotional contagion study and the ethical problem of co-opted identity in mediated environments where users lack control. Research Ethics, 12(1), 35–43. https://doi.org/10.1177/1747016115579531
- Thépot, F. (2013). Market power in online search and social networking: A matter of two-sided markets. World Competition, 36(Issue 2), 195–221. https://doi.org/10.54648/woco2013015
- Zuboff, S. (2019). The age of surveillance capitalism: The fight for the human future at the New Frontier of Power. Profile Books.