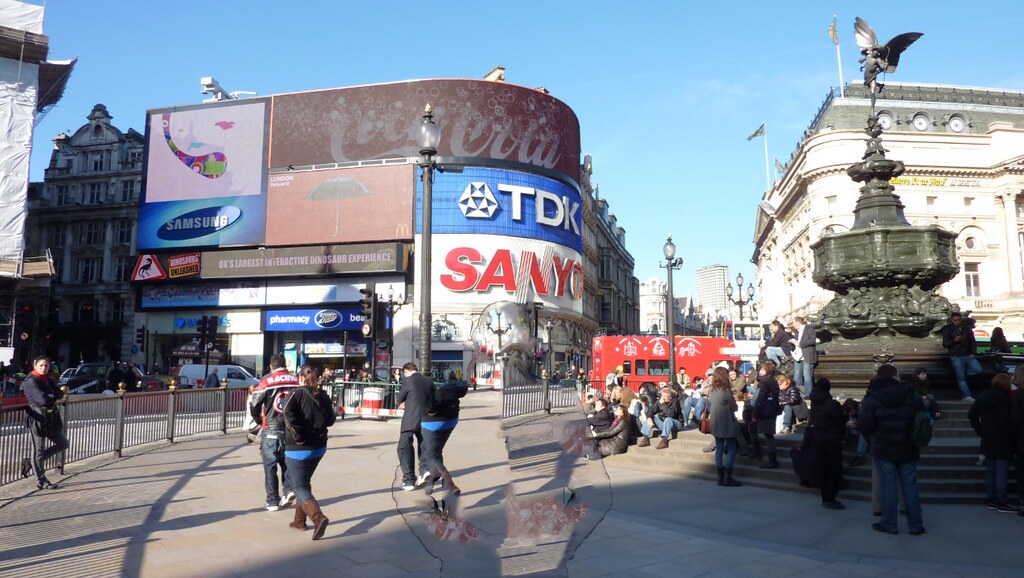
“content aware fill fail” by Sean MacEntee is licensed under CC BY 2.0.
The Rise of Algorithmic Influence in the Digital Age
In today’s digital age, the internet and social media platforms have evolved into an era of unprecedented connectivity and communication. However, with change comes a challenge – significant transformation in how the community shares ideas and information has raised a pressing issue on algorithmic biases. Algorithms have become a highly influential and potent tool in social media nowadays. They exert control over several aspects including societal, economic, and political domains – extending their influence on the personal lives of individuals. Social scientists have emphasized that algorithms are not merely mathematical or computational procedures; instead, they view them as complex socio-technical instruments responsible for generating knowledge and serving as an essential tool in critical social decision-making. Nevertheless, it is important to acknowledge that algorithms are susceptible to bias, defined as a replicable error (Iwasiński, 2020). This article will dive deep into the intricacies of algorithmic biases, focusing on the processes of shadowbanning and content rule enforcement. These biases are not technical glitches but potent forces that have profound implications for the fundamental principle of free expression. Moreover, they serve as conduits through which system inequalities persist in our increasingly digitalized world.
Shadowbanning: Origins and Controversies
Shadowbanning represents a contentious form of content moderation within the realm of social media. It was first coined by moderators on the Something Awful website and online forum in 2001, involving the discreet action of concealing posts from all users except the account holder. This approach creates the users being unaware of the ban, therefore continuing to interact with what appears to be an audience (Savolainen, 2020). In 2018, shadow banning got even more public recognition when accusations surfaced, some suggest that Twitter was implementing this technique against ‘prominent Republicans’. Former U.S. President Donald Trump was even weighed on the issue, labeling the moderation tactic as ‘illegal’, other cases like Alex Jones ban on YouTube have gained debate and public reactions. A variety of responses have arisen about shadow banning. Some dismiss them as unfounded paranoia, attributing them to misunderstandings about how platforms curate content. However, others acknowledge the existence of shadow banning but remain divided on its ethical implications (Leerssen, 2023).
Examining Content Exposure Perspectives
The concept of content exposure can be seen from two angles: an active perspective – which involves what users are allowed or prohibited to post and share on their accounts, and a passive perspective – which concerns what users encounter or do not encounter when using social media. Currently, the active perspective is subject to regulation through community rules, focusing on prohibiting various content types – such as hate speech, terrorist content, and other problematic issues. In contrast, the passive perspective has received less attention and remains largely unregulated (Stasi, 2019). The issue of content exposure demands significant attention, particularly because information goods play a pivotal role in society. As such, this essay contends that algorithmic biases, particularly evident in shadowbanning and content rule enforcement, pose a significant threat to the principle of free expression and perpetuate systemic inequalities in the digital age.
Algorithmic Biases in YouTube’s Recommendation System
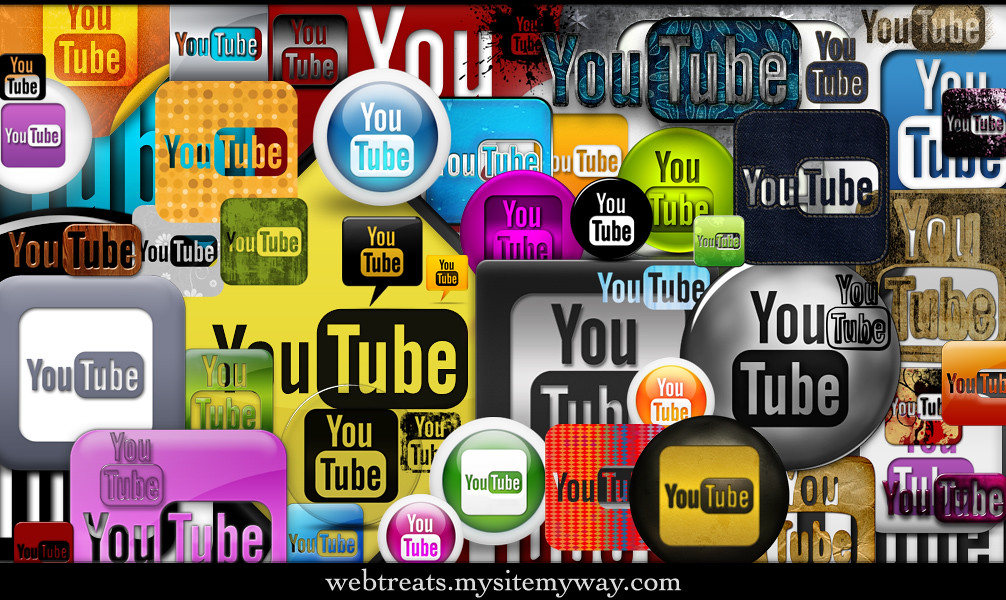
“Webtreats – 272 YouTube Icons Promo Pack” by webtreats is licensed under CC BY 2.0.
YouTube, one of the world’s biggest video-sharing platforms, relies heavily on algorithms to recommend videos to its users. These algorithms consider various factors, including a user’s interaction history. While the intention behind this algorithm is to enhance user experience and retain users on the platform, it has come under intense scrutiny and criticism.
Concerns have arisen regarding algorithmic biases with YouTube’s recommendation system. The algorithm’s inclination to suggest content similar to what a user has previously engaged with can result in the promotion of extreme or polarizing content. As an example, if a user watches a politically oriented video, the algorithm might recommend more politically extreme content, thereby reinforcing existing biases and creating echo chambers. Criticism has also been directed at this recommendation algorithm for its role in amplifying misinformation, conspiracy theories, and extremist viewpoints. Users have reported instances when they initially engaged with harmless content but were gradually directed towards more radical or misleading material due to the algorithm’s recommendations. This example highlights how algorithmic biases, even with well-intentioned systems can lead to unintended consequences, influencing user behavior and the dissemination of information.
YouTube’s primary goal has been profit generation rather than education or information dissemination. Hence, this profit-oriented approach has led to concerns about the platform’s neutrality and its ability to serve as an impartial source of information. Now without regulation, certain users with specific agendas can exploit the algorithm to control content presentation. This manipulation includes Search Engine Optimization (SEO) tactics and alignment with political structures (Bryant, 2020).
Algorithmic Influence on Content Rule Enforcement
Shadowbanning involves suppressing or limiting the visibility of certain users or content without their knowledge, often based on their past behavior or content violations. In this case, algorithms play a role in shaping the content users are exposed to, and this can unintentionally amplify or restrict certain types of content. So, even well-intentioned algorithms can have unintended consequences on user behaviour and content dissemination, which aligns which the concerns of shadowbanning.
With YouTube’s profit-driven approach and the potential for users to exploit the algorithm to control content presentation relates to content rule enforcement. Content rule enforcement on platforms involves moderating content to ensure it complies with community guidelines and standards. However, profit motives and algorithmic biases can influence how content rule enforcement is carried out. Platforms may prioritize content that generates higher engagement, potentially leading to the promotion of extreme or polarizing material as mentioned in earlier passage. This raises questions about the effectiveness of content rule enforcement, highlighting the need for transparency and accountability in managing algorithms that govern content recommendations and enforcement on digital platforms.
Content Choice and Algorithms: A Delicate Balance
The concept of freedom of content choice in the context of digital algorithms is a double-edged sword. On one hand, algorithms are designed to tailor content recommendations for individual preferences, providing users with a personalized and engaging experience. Hence, this level of personalization can allow the user to discover content that aligns with their interests and preferences. Users are no longer limited to a one-size-fits-all approach to content consumption.
However, the concern arises when the level of personalization becomes too narrow, potentially leading to filter bubbles and echo chambers. Filter bubbles occur when users are exposed only to content that reinforces their existing beliefs and perspectives. This can lead to detrimental effects on society, as it limits exposure to diverse viewpoints and can contribute to polarization. For instance, if a user predominantly watches content from one political ideology, the algorithm may continuously recommend similar content, reinforcing the user’s existing beliefs and shutting out alternative perspectives.
To address this concern, platforms should strike a balance between personalization and diversity. While algorithms can cater to individual preferences, they should also incorporate systems that expose users to a variety of viewpoints and topics. This approach not only respects user’s freedom of content choice but also promotes a more informed and open-minded digital society.
Although mentioned earlier YouTube is a profit-driven business which has negative connotations, YouTube relying on advertising revenue sustains its operations and provides free access to users. This model has allowed content creators from all walks of life to share their work with a global audience, democratizing content creation and distribution. However, the profit motive can sometimes clash with the goal of serving as a neutral and impartial source of information. Platforms may prioritize content that generates higher engagement and, consequently, more advertising revenue. This can lead to the promotion of sensational content which does not promote balanced information.
This is where content rule enforcement comes in, to strike a balance between profit and responsibility – where digital platforms must consider ethical and transparency measures. By doing so, platforms can uphold their business sustainability while also fulfilling their role as responsible information providers.
Algorithmic Influence and Content Control: Challenges in the Digital Age © 2023 by Emily Sidanta is licensed under CC BY 4.0
Reference List
Social Implication of Algorithmic Bias (December 2020.). ResearchGate | Find and share research. (PDF) SOCIAL IMPLICATIONS OF ALGORITHMIC BIAS
The shadow banning controversy: perceived governance and algorithmic folklore. (2022, March). Sage Journals. https://doi.org/10.1177/01634437221077174
An end to shadow banning? Transparency rights in the Digital Services Act between content moderation and curation. (2023, April). sciencedirect.com. https://doi.org/10.1016/j.clsr.2023.105790
Social media platforms and content exposure: How to restore users’ control. (2019, June 9). Sage Journals. https://doi.org/10.1177/1783591719847545
Search engine optimization. (2023, September 13). Wikipedia, the free encyclopedia. Retrieved September 29, 2023, from Search engine optimization – Wikipedia
The YouTube Algorithm and the Alt-Right Filter Bubble. (2020, June). ResearchGate | Find and share research. https://doi.org/10.1515/opis-2020-0007