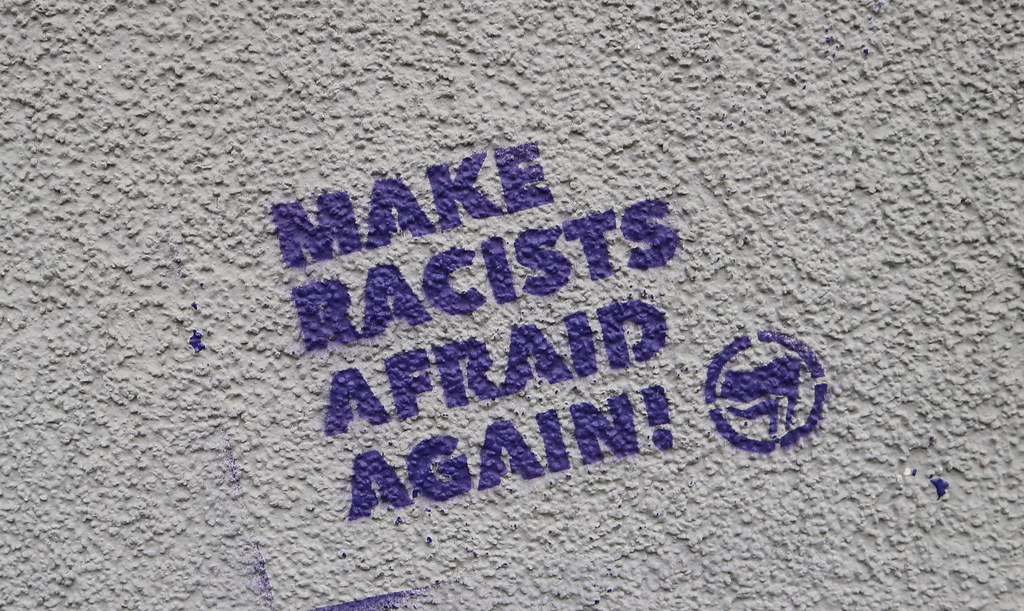
Introduction
Platforms, as tools for information dissemination, should effectively regulate user-produced content as a way to maintain social order and protect the legitimate rights of users. However what sometimes seems utopian, the dangers are also evident, which include: pornography, obscenity, violence, abuse and hate bias (Gillespie, 2019). There are multiple aspects to this issue, including platform policy censorship and algorithmic bias.
Bias in platform monitoring algorithms
Algorithms are widely used in major platforms, which helps to increase the efficiency of humans in accomplishing diverse information processing tasks. This also provides users with services such as personalised recommendations, information customisation and autonomous decision-making. Although algorithms are sometimes considered neutral and objective, many studies in recent years have shown that algorithm-induced bias extends to the Internet.
The Information Cocoon
Modern data-media algorithms analyse user data, which means that social platforms like TikTok, based on “personalisation” algorithms, provide a new dimension for matching content to users. Personalisation algorithms highlight individual preferences, and each user’s feed is unique and marked as “For you”. This goes some way to reducing the cost of being a consumer of information and means that what the user sees is handpicked by the algorithm and provides the user with similar content. This is what Sunstein (2008) refers to as the “information cocoon”, where the public’s own information needs are not all-encompassing, and the public only pays attention to what it chooses to see and to the areas of information that delight it.
In the Internet generation, people’s ability to process information is limited. Therefore, people have to make choices among the massive amount of information. In other words, in order to attract the attention of the audience, the platform will provide corresponding information for specific audience interests and characteristics, which undoubtedly further strengthens the audience’s original inherent information. The audience will pay more attention to their own closed information circle, and gradually create an information cocoon for themselves. As a result, individuals and groups become more polarised, considering their own prejudices as the truth, thus rejecting the integration of other viewpoints, and even launching challenges and attacks on the platform.
Perception
Platform algorithms have absolute power to control the information users see, and such biased algorithms will lead to information inequality.
At the same time inherently human-biased algorithms reproduce socio-structural inequalities, further reinforcing the inequality and underclass position that disadvantaged groups occupy in digital media.
This also demonstrates that algorithms reproduce the socio-political and cultural structures present in white patriarchal societies (Papakyriakopoulos & Mboya, 2022).
Whereas algorithmic bias is invisible, traditionally disadvantaged groups are marginalised in the platform’s algorithms. This can be seen, for example, in the following video:
A TikTok user was censored by the platform after attempting to add the phrase “Black Lives Matter” on his platform. TikTok creator Ziggi Tyler, who has more than 370,000 followers, has attempted a number of phrases about black people and has been prompted by the platform to “remove any inappropriate content”. However, when words such as “supporting white supremacy” were added, the results showed that this did not trigger the platform’s penalty mechanism.
This is significant evidence that algorithmic bias leads to algorithmic limitations for those non-vulnerable or popular groups who may also lack algorithmic bias perception, and in the long run, their perceptions are controlled by the algorithm in a cocoon, even contributing to antagonism.
Radical Algorithms for Platform Monitoring
Due to the combination of algorithmic principles of media platform monitoring and gendered interactions, users are often controlled by algorithms to see bifurcated content (Grandinetti & Bruinsma, 2022). The extreme tendency for algorithms to behave differently based on personalized information is even more pronounced.Social media, such as TikTok, are attracted to users by fostering an emotional attachment that manifests itself in love, loyalty, and habituation to the platform (Grandinetti & Bruinsma, 2022).
The platform’s algorithms are influenced by user interactions, video messages, and the settings of accounts, according to TikTok’s policy display . And it claims to be committed to protecting users from conspiracies of false or extreme information. Yet these promises run counter to the fact that relevance and connectivity are paramount on the platform (Grandinetti & Bruinsma, 2022).
A study suggests testing by creating a new fictional teen account. Initially, video content included comedy, animals, and discussions about male mental health. However, after watching videos aimed at male-oriented users (included male mental health), the platform’s algorithms began to recommend more content that seemed to be tailored to men. And these displays occur without the user actively commenting or searching, long after the video content also includes accusations of feminist and misogynistic views. This demonstrates the spread of hate speech and extremist content in the content suggested by the algorithm (Grandinetti & Bruinsma, 2022).
Inequality in the platform’s monitoring structure
Research on social media suggests that the ideals claimed by digital platforms of presenting broader or fairer gender constructions are often not realized (Singh et al., 2020).
Platforms’ search engines also typically perpetuate gender stereotypes that intersect with other identity categories (e.g., race, class, disability) and related inequalities (Singh et al., 2020).
The PageRank used by the Google platform generates results that frequently correlate Black women () with pornographic content. Google image search results for a variety of occupations systematically underrepresent women (Singh et al., 2020). Google frequently associates stereotypes and and negativity with non-White people and favors descriptions of adjectives of appearance and physical features. However these traditional stereotypes are not monitored by Google. This also suggests that pre-existing gendered power relations introduce inequality into technological systems with enough influence to produce stereotypical messages and images (Singh et al., 2020).
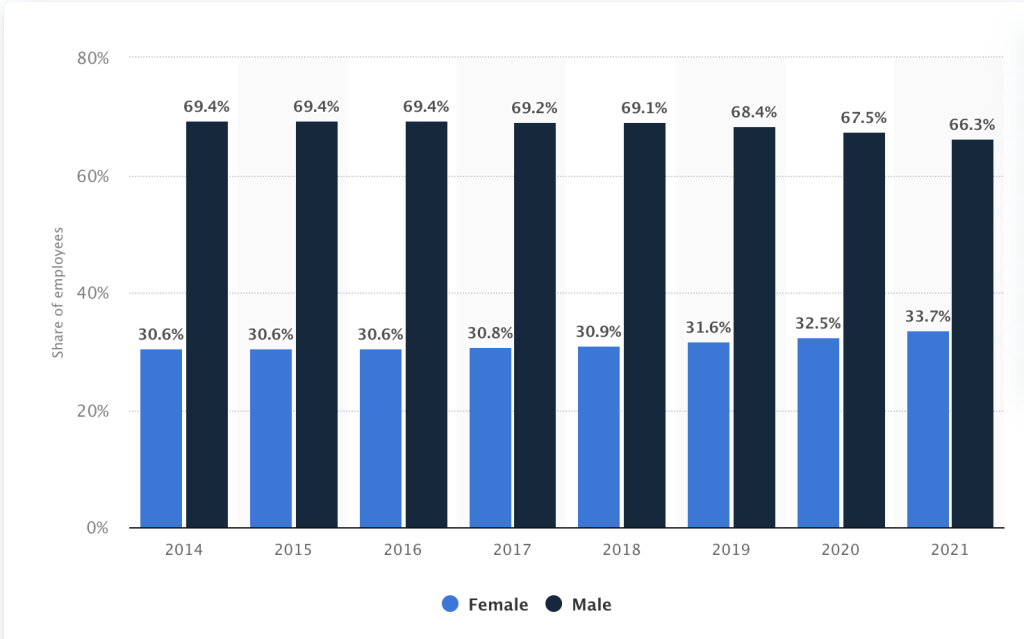
In other words, this unfairness relies on the inequality of the traditional social structure, which gives the privileged class an absolute advantage in terms of voice control and social resources (e.g. employment opportunities). As the statistics below show, there is a very large gap between the proportion of male and female employees at Google, with men dominating the majority. Such unfair distribution exacerbates the exclusion of groups that are already in a socially disadvantaged position.
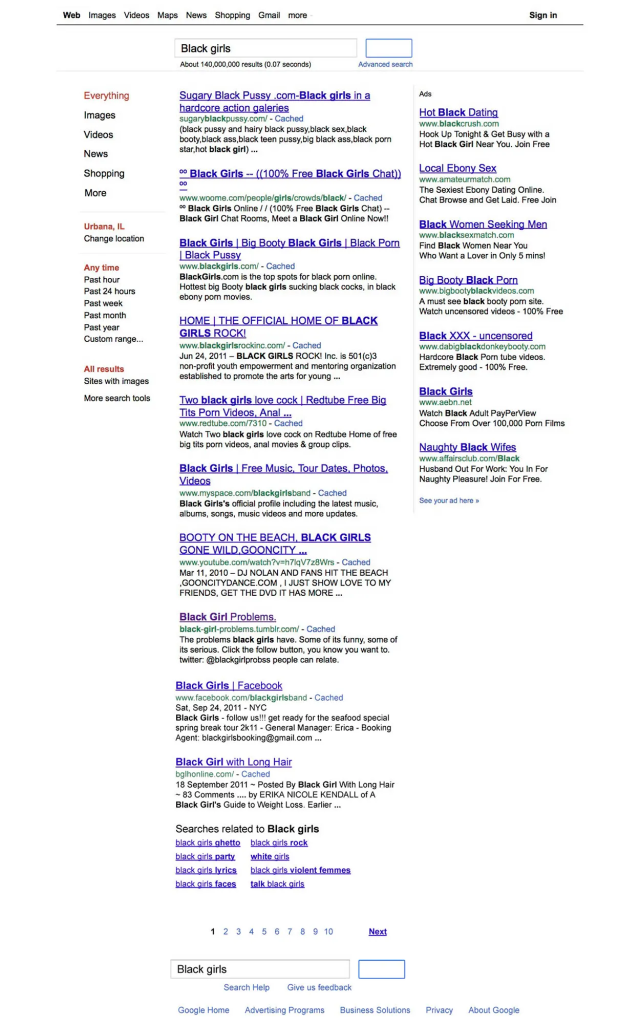
This disparate structure crystallizes a specific inherent culture and continues to deepen the minds of users who use search engines. Although sometimes this bias stems from problematic user queries, the responsibility for discriminatory censorship reasoning remains with the technology owners and designers (Papakyriakopoulos & Mboya, 2022).
Thus, it is demonstrated that platform staff are the ones higher up the hierarchical ladder who create biased representation by placing their inherent culture within the platform’s norms of surveillance (Singh et al., 2020).
Future Monitoring Expectations
The expectation is that monitoring on social platforms is transparent and open, with platforms clearly indicating their content censorship policies and how their algorithms work.
In addition, the monitoring platform’s staff should be structured in a way that is fair and does not favor any particular political viewpoint or interest.
Monitoring organizations are expected to promote user advocacy and digital literacy to help users better understand how to use social media platforms.
Committed to working together to build an open, shared and inclusive Internet environment
Reference
Gillespie, T. (2019). All Platforms Moderate. In Custodians of the Internet: : Platforms, Content Moderation, and the Hidden Decisi (pp. 1–23). Yale University Press. https://doi.org/10.12987/9780300235029
Google: gender distribution of global employees 2019 | Statista. (2019). Statista; Statista. https://www.statista.com/statistics/311800/google-employee-gender-global/
Grandinetti, J., & Bruinsma, J. (2022). The Affective Algorithms of Conspiracy TikTok. Journal of Broadcasting & Electronic Media, 67(3), 1–20. https://doi.org/10.1080/08838151.2022.2140806
Kung, J. (2022, February 14). What internet outrage reveals about race and TikTok’s algorithm. NPR. https://www.npr.org/sections/codeswitch/2022/02/14/1080577195/tiktok-algorithm
Papakyriakopoulos, O., & Mboya, A. M. (2022). Beyond Algorithmic Bias: A Socio-Computational Interrogation of the Google Search by Image Algorithm. Social Science Computer Review, 089443932110731. https://doi.org/10.1177/08944393211073169
Singh, V. K., Chayko, M., Inamdar, R., & Floegel, D. (2020). Female Librarians and Male Computer Programmers? Gender Bias in Occupational Images on Digital Media Platforms. Journal of the Association for Information Science and Technology. https://doi.org/10.1002/asi.24335
Sunstein, C. R. (2008). Infotopia : how many minds produce knowledge. Oxford University Press.