The ideals of the ‘open’ and ‘free’ platforms that resonate with democratic and utopian principles are fallacies; platforms must moderate content in one way or another (Gillespie, 2018).
With the increasing proliferation of social media and the evolving nature of its affiliated affordances, platform moderation emerges as an ever-challenging pursuit to control user-generated content. In the face of mounting governmental pressures, legal liability and public expectations, heightened demands for platform responsibility and security constitute focal areas of concern for tech conglomerates. Within this context, the key characteristics of social networking platforms – their sheer magnitude and reach – suggest that artificial intelligence (AI) represents the only pragmatic solution to scalable content moderation (Gillespie, 2020). Accordingly, this analysis will first explore the challenges intrinsic to human content moderation and, second, present the arguments that advocate a transition to AI-based moderation as a prospective panacea to mitigate these challenges. Finally, despite the potential promise of what Gorwa (et al., 2020) define as algorithmic moderation systems, these systems remain in nascent development stages and are consequently riddled with deficiencies. As such, this analysis will undertake an examination of the issue of algorithmic bias entailed in the deployment of AI-based content moderation.
The Challenges of Human Content Moderation
The paramount challenge for online platforms is the delineation of the thresholds distinguishing ‘acceptable’ from ‘unacceptable’ content. Accordingly, it is essential to recognise the inherent fluidity of the very concept of acceptability. Norms dictating what qualifies as acceptable or unacceptable content exhibit variance across different online platforms, and a universal consensus on the definition of such content remains elusive. For instance, X (formerly known as Twitter) has come to be perceived as prioritising freedom of expression over content previously prohibited on the platform, a shift that transpired after its change in ownership to Elon Musk. A 2023 New York Times article reported that Musk has now reversed policies that banned openly racist and anti-Semitic users “under the aegis of free speech” (Walsh, 2023).
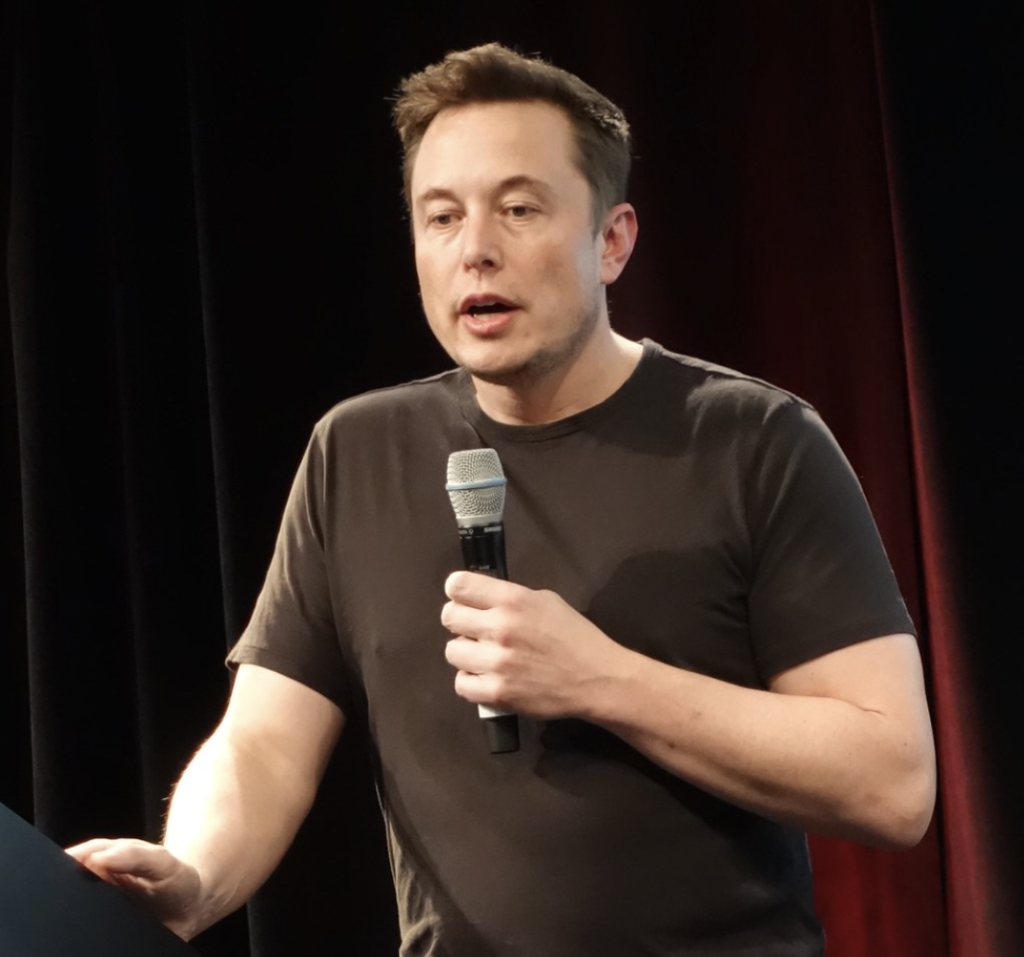
The lack of universal principles for content moderation complicates the task. Content moderation demands immense human resources, relying upon human judgement and subjectivity to adjudicate content’s appropriateness and decide whether it needs to be removed.
In addition to the inconsistency of the principles underpinning content moderation, the labour undertaken by these individuals takes place almost covertly, with the roles marked by meagre remuneration and unfavourable working conditions (Barnes, 2022). Nonetheless, with increased journalistic scrutiny, leaks, and increasing examples of public opprobrium arising from failed moderation, the intricacies of human content moderation are emerging into the public consciousness.
A significant illustration of these challenges is illuminated in a 2021 YouTube video published by VICE titled ‘The Horrors of Being a Facebook Moderator’. The story featured an anonymous individual who detailed the abhorrent and traumatic content that Facebook moderators are routinely exposed to. Such content goes far beyond that which might be politically unpalatable – it covers content considered illegal in every circumstance. The reporting highlighted that relatively untrained and unsupported moderators were required to make complex moral and ethical decisions about the appropriateness of such content. They disclosed that Facebook imposed stringent nondisclosure agreements (NDAs) and emphasised the prohibition against discussing their employment with anyone. Only once they left the role did the anonymous worker begin to comprehend the profound mental toll exacted by their moderation work and consequently receive a diagnosis of post-traumatic stress disorder (PTSD). This account served as a compelling exposé, shedding light on the harsh realities of human content moderation and the associated mental health challenges.
VICE, 2023
In addition to the ethical concerns regarding human moderators, the prolific increase in content posted daily to mainstream social media platforms renders manual content moderation increasingly unfeasible and impractical. Gillespie (2020, p. 1) contends that as social networking platforms and userbases have grown, “so has the problem of moderating them”, with demands to disperse the workforce globally in response to the incessant need for monitoring (Roberts, 2019).
A False Dawn – AI Content Moderation?
Mega-platform companies presently operate at such a scale that there is a demand to move away from traditional manual forms of content moderation and towards alternative methods to address existing deficiencies. Incidents such as the 2016 US Presidential Election, Gamergate and the Christchurch massacre indicate that the repercussions of online harms reverberate beyond the confines of the platform, and thus, the pressures on the content platforms to rectify the moderation failures have intensified (Gillespie, 2020). Against the backdrop of profound technological advancements in machine learning, AI has emerged as “the force” that is said to have the means to alleviate the issues inherent within moderation (Gorwa et al., 2020, p. 2).
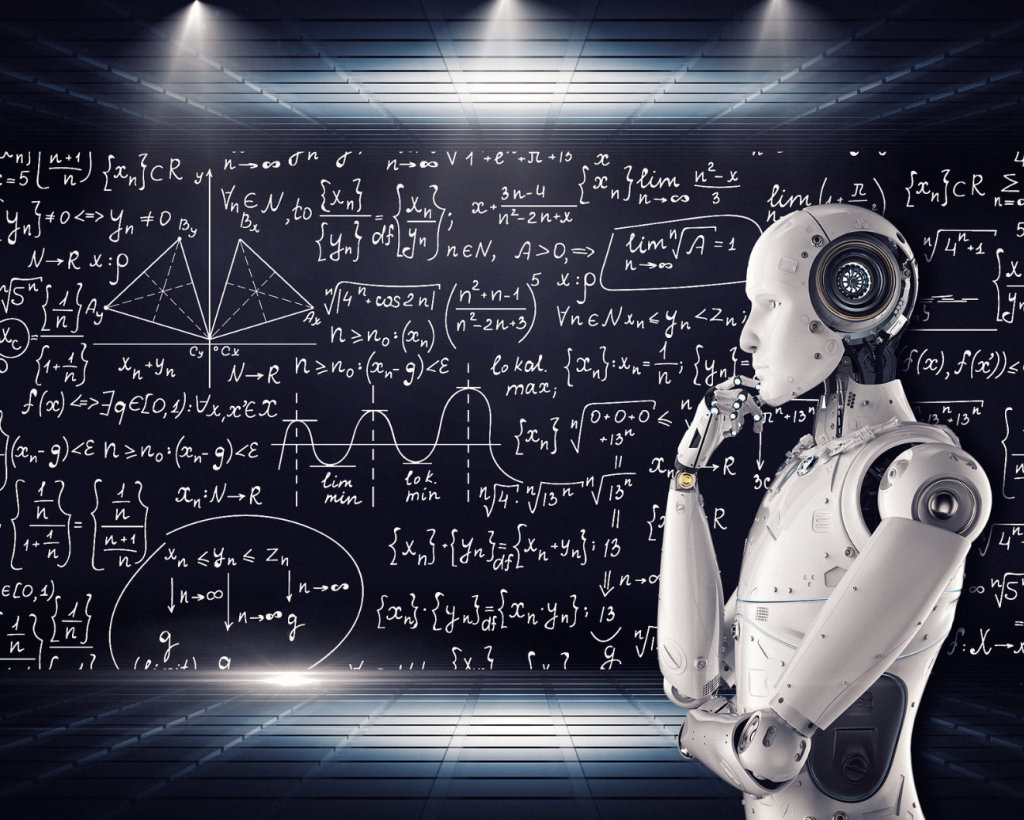
With increasing governmental pressure, major tech platforms are deciding (whether under legal compulsion or otherwise) to establish more effective and efficient systems for content evaluation. For instance, a 2016 article by The Guardian reported on the European Union’s development of a code of conduct to fight hate speech in conjunction with four of the world’s biggest internet companies (Hern, 2017). The proposed code set out a range of commitments to be undertaken by the platforms, including mandating the removal of illegal hate speech in less than 24 hours. The stringent timeframes enforced by the government, combined with the volume of content, ultimately necessitate the use of automated systems to detect problematic material “proactively and at scale” (Gorwa et al., 2020, p. 2).
The current models of AI-based content moderation seek to identify, match, predict or classify a piece of content, such as video, text, audio or image, on “the basis of its exact properties or general features” (Gorwa et al., 2020, p. 3). These tools are then deployed to police content across several issues at scale (e.g. terrorism, hate speech, child pornography, spam accounts). Several social networking platforms have already incorporated elements of AI into their content moderation practices. For instance, Facebook uses machine learning algorithms to flag and remove potential content supporting terrorist groups (Gorwa et al., 2020). These systems are trained on a corpus of data to create predictive scores to determine how likely a post is to violate Facebook’s terrorism policies and have led to a massive increase in the number of terrorism-related takedowns. Table 1 presents statistics demonstrating improvements to Facebook’s algorithmic content moderation across 2018. Notably, in Q2 2018, Facebook reported removing 9.4 million pieces of ISIS and al-Qaeda content. Additionally, the amount of time terrorist content reported by users remains on the platform reduced from 43 hours in Q1 of 2018 to 18 hours in Q3 (Bikert & Fishman, 2018).
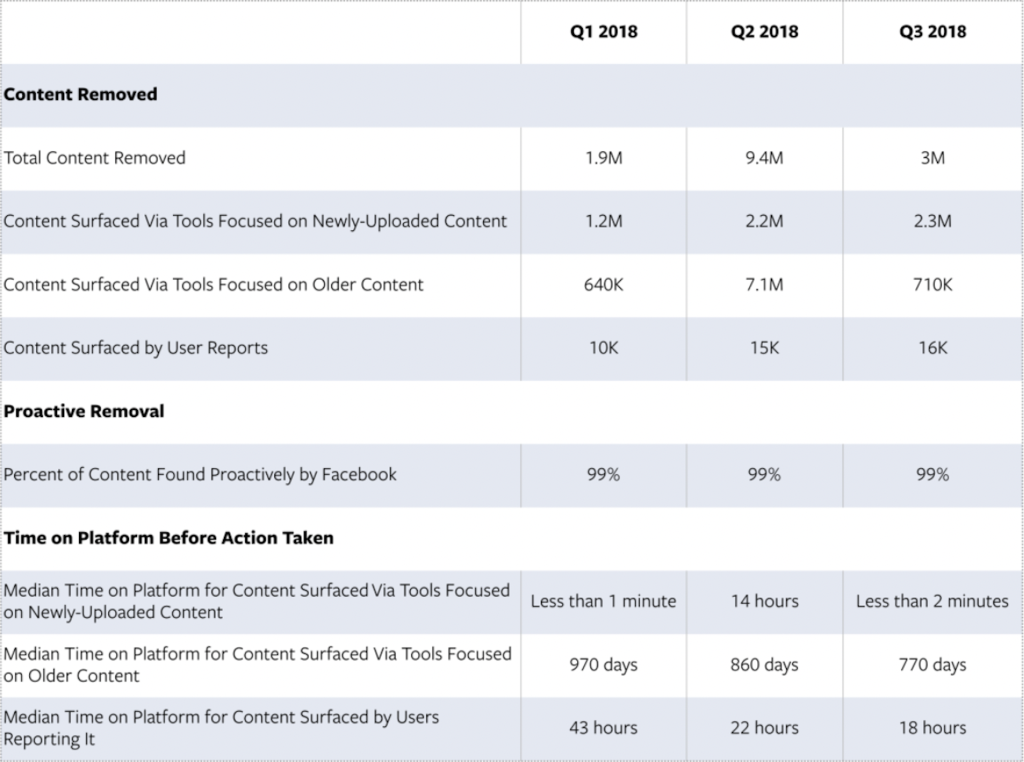
Table 1: Bikert & Fishman, 2018
In the case of Facebook, algorithmic content moderation has effectively identified and removed terrorist-related content and significantly reduced the time for which that content remains on the platform.
AI-Based Content Moderation: The Thorn of Algorithmic Bias
Despite suggestions that algorithmic content moderation is necessary to manage heightened societal and governmental expectations about increased platform responsibility and safety, it is crucial to underscore that the existing systems are opaque, obscure and riddled with accountability issues. Notably, Gorwa (et al., 2020, p. 1) contend that AI-based content moderation may paradoxically “exacerbate, rather than relieve”, many existing problems with the content policy. Algorithmic bias poses a formidable challenge within the realm of AI-based content moderation. Despite the prevailing assumption that algorithms, underpinned by vast datasets, operate with objectivity and neutrality, they are, in practice, susceptible to encoding and perpetuating discriminatory practices (Noble, 2018).
This process has brought to light a disconcerting revelation: AI-based content moderation procedures on digital platforms “largely reflect U.S.-based norms”, thereby accentuating the integration of prejudicial ideas into the very fabric of the moderation framework (Noble, 2018, p. 57). This proclivity for AI to replicate bias has already manifested itself in critical domains, including criminal justice, where it has been employed to assess individuals with prior criminal convictions. Significantly, the Correctional Offender Management Profiling for Alternative Sanctions (COMPAS) system used data from a cohort of male offenders released under parole supervision to identify individuals who are likely to recidivate (Herrschaft, 2014). However, a 2016 report from ProPublica found that COMPAS was inclined to mistakenly classify black offenders as twice the rate more likely to re-offend than white offenders. With racial bias encoded in the COMPAS algorithms, a disproportionate number of black people were labelled as ‘high risk’ when, in fact, they did not re-offend (Angwin et al., 2016).
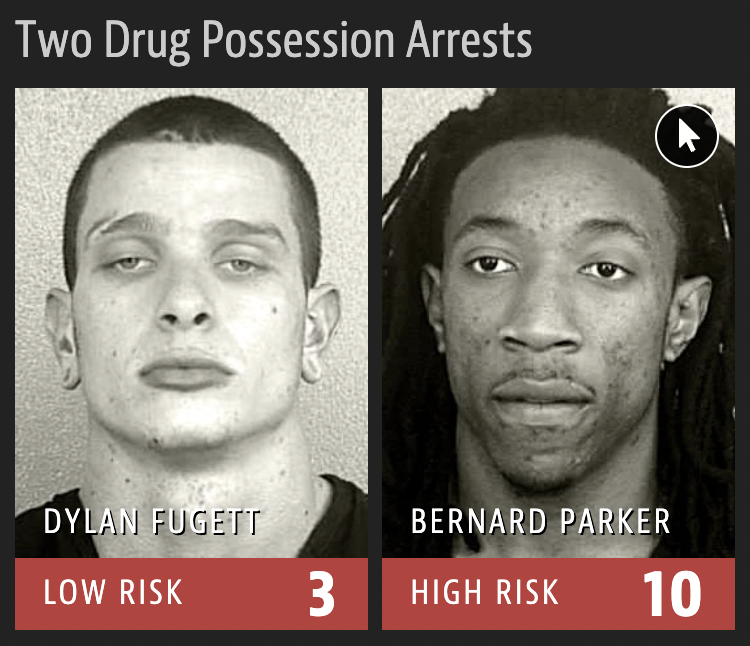
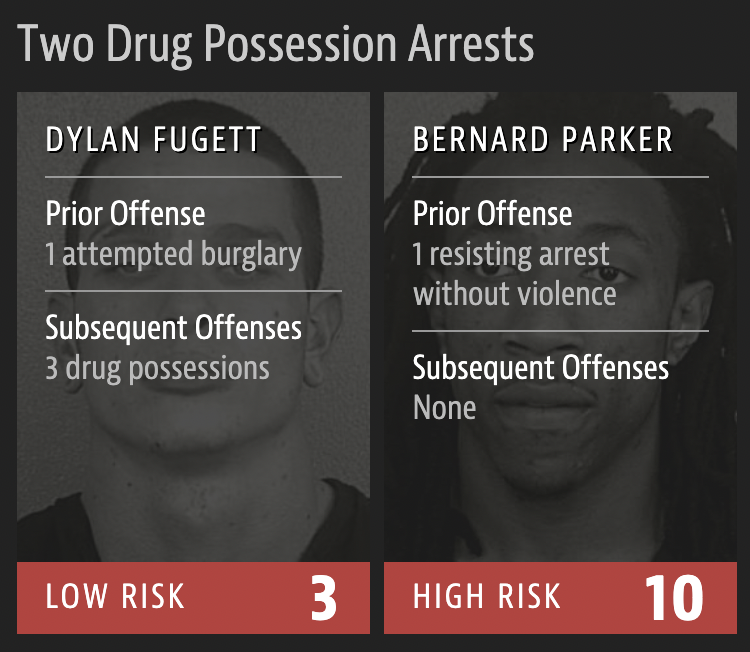
Angwin et al., 2016
This case is a poignant illustration of AI’s tendency to replicate bias, thereby underscoring the extent to which “technical schemas enforce hierarchies and amplify inequities” (Crawford, 2021, p. 17).
Parallel concerns reverberate concerning algorithmic content moderation, where content classifiers may exhibit bias towards protected categories under anti-discrimination laws. Even algorithms designed to accurately identify ‘toxic’ speech are inherently predisposed to prioritise certain interpretations of offence over others, consequently introducing bias into the content moderation process (Gorwa et al., 2020). An illustrative case is Facebook’s well-intentioned effort to establish policies that purported to treat all racial categories equally yet failed to address the disproportionate impact of racial discrimination across much of the Global North. As such, these policies failed to carve out specific hate speech protections for particular ‘sub-categories,’ such as ‘black children’ (Gorwa et al., 2020).
In this context, algorithmic bias becomes acutely manifest, as automated decision-making mechanisms risk exacerbating preexisting bias rather than alleviating it (Ajunwa, 2020). It is imperative to recognise that removing human moderators from the decision-making equation does not guarantee impartiality. In fact, it often engenders a paradoxical situation wherein automated content moderation systems inadvertently perpetuate and amplify existing biases (Ajunwa, 2020, p. 1673).
“The dream of automating content moderation is often justified as a response to scale. The immense amount of the data, the relentlessness of the violations, the need to make judgments without demanding that human moderators make them, all become the reason why AI approaches sound so desirable, inevitable, unavoidable to platform managers”
Gillespie, 2020, p. 2
Reference List
Ajunwa, I. (2020). The Paradox of Automation as Anti-bias Intervention. Cardozo Law Review, 41(5), 1671–.
Angwin, J., Larson, J., Mattu., S & Kirchner, L. (2016). Machine Bias: There’s software used across the country to predict future criminals. And it’s biased against blacks. ProPublica. Available at:
https://www.propublica.org/article/machine-bias-risk-assessments-in-criminal-sentencing
Barnes, M.R. (2022). Online Extremism, AI, and (Human) Content Moderation. Feminist Philosophy Quarterly, 8(3/4).
Bikert, M & Fishman, B. (2018) Hard questions: What are we doing to stay ahead of terrorists? Facebook Newsroom. Available at: https://perma.cc/YRD5-P5HU
Binns, R., Veale, M., Van Kleek, M., & Shadbolt, N. (2017). Like Trainer, Like Bot? Inheritance of Bias in Algorithmic Content Moderation. In Lecture Notes in Computer Science (including subseries Lecture Notes in Artificial Intelligence and Lecture Notes in Bioinformatics) (Vol. 10540, pp. 405–415). Springer International Publishing. https://doi.org/10.1007/978-3-319-67256-4_32
Crawford, K. (2021). Atlas of AI : Power, Politics, and the Planetary Costs of Artificial
Intelligence. Yale University Press.
https://doi.org/10.12987/9780300252392
Gillespie, T. (2018). Custodians of the Internet : Platforms, Content Moderation, and the Hidden Decisions That Shape Social Media. Yale University Press.
https://doi.org/10.12987/9780300235029
Gillespie, T. (2020). Content moderation, AI, and the question of scale. Big Data & Society, 7(2), 205395172094323–.
https://doi.org/10.1177/2053951720943234
Gorwa, R., Binns, R., & Katzenbach, C. (2020). Algorithmic content moderation: Technical and political challenges in the automation of platform governance. Big Data & Society, 7(1), 205395171989794–.
https://doi.org/10.1177/2053951719897945
Hern, A. (2017, November 28). Facebook, YouTube, Twitter and Microsoft sign EU hate speech code. The Guardian.
Herrschaft, B, A. (2014). Evaluating the reliability and validity of the Correctional Offender Management Profiling for Alternative Sanctions (COMPAS) tool.
https://doi.org/doi:10.7282/T38C9XX2
Llansó, E. J. (2020). No amount of “AI” in content moderation will solve filtering’s prior-restraint problem. Big Data & Society, 7(1), 205395172092068–.
https://doi.org/10.1177/2053951720920686
Mukherjee, A. (2023). AI and ethics : a computational perspective. IOP Publishing.
https://doi.org/10.1088/978-0-7503-6116-3
Noble, S. U. (2018). Algorithms of oppression : how search engines reinforce racism. New York University Press.
Roberts, S. T. (2019). Behind the screen : content moderation in the shadows of social media. Yale University Press.
https://doi.org/10.12987/9780300245318
VICE. (2021). The Horrors of Being a Facebook Moderator | Informer [Video file]. YouTube. https://www.youtube.com/watch?v=cHGbWn6iwHw
Walsh, D. A. (2023, September 11). Opinion | Elon Musk Has Crossed a Line. The New York Times. https://www.nytimes.com/2023/09/11/opinion/elon-musk-adl.html