1. Introduction &Thesis Statement
In the modern age of technology, search engines have become our primary gatekeepers of information. However, what happens when these gatekeepers carry biases that influence public perception? This short hyper-textual essay explores the harmful biases present in search engines and their implications for society. This essay argues that search engines, despite appearing neutral, manifest biases that shape user perception, perpetuate stereotypes, and exacerbate societal divisions.
2. Supporting Evidence, Analysis and Discussion
2.1 The Algorithms Behind the Bias
At the core of search engines are algorithms that determine the ranking and relevance of search results. While these algorithms aim for accuracy and user satisfaction, they can inadvertently favor certain sources or perspectives. Furthermore, because these algorithms rely on user data and past search histories, they often create “filter bubbles,” wherein users are only exposed to information that aligns with their existing beliefs.
Take, for instance, complications arise when algorithms prioritize content based on a user’s past search history, clicks, and geographical location. In 2016, researchers from the University of Amsterdam analyzed the Google search results of various individuals and found that personalized results varied significantly based on the digital profiles of users (As shown in Graph. 1). Additionally, as revealed by the study ‘An Investigation of Biases in Web Search Engine Query Suggestions’ conducted by Bonart et al. (2019), This highlights a broader challenge known as the “filter bubble” phenomenon, coined by internet activist Eli Pariser. Essentially, as search engines strive to cater to individual preferences, they trap users in a feedback loop, continuously presenting them with content that aligns with their pre-existing beliefs and interests.
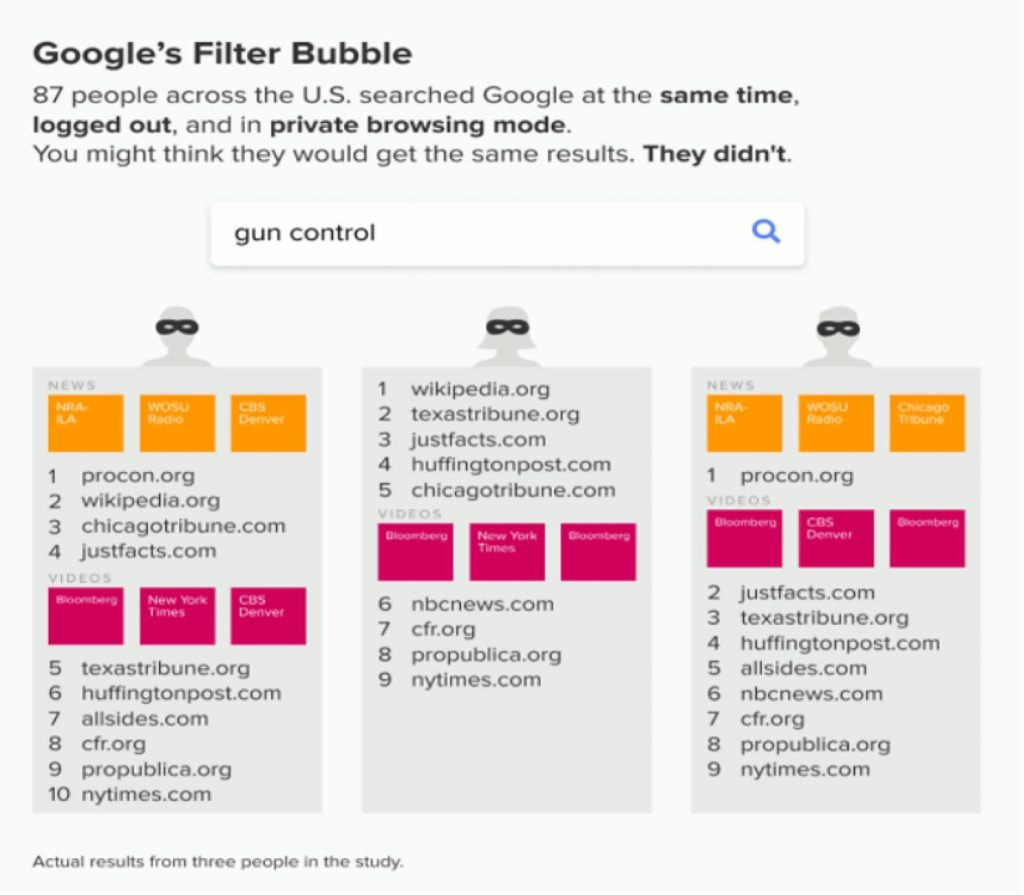
Graph 1: the “Filter Bubble”: How Google is influencing what you click, licensed under spreadprivacy.com
(Source: https://spreadprivacy.com/google-filter-bubble-study/.,2023)
For a tangible understanding of filter bubbles, consider a user who frequently reads conservative news outlets and searches for conservative political commentary. Over time, their search results will lean heavily towards conservative viewpoints, even if they input neutral or broad political queries. Conversely, someone with a history of engaging with liberal content will find their results populated primarily by left-leaning sources, as indicated in the research of “Algorithmic Gatekeeping for Professional Communicators: Power, Trust, and Legitimacy.” conducted by van Dalen (2023). The implication is clear: two individuals can search for the same term, like “climate change,” and receive markedly different content on social media platforms (Graph 2), reinforcing their existing beliefs rather than offering a diverse range of perspectives.
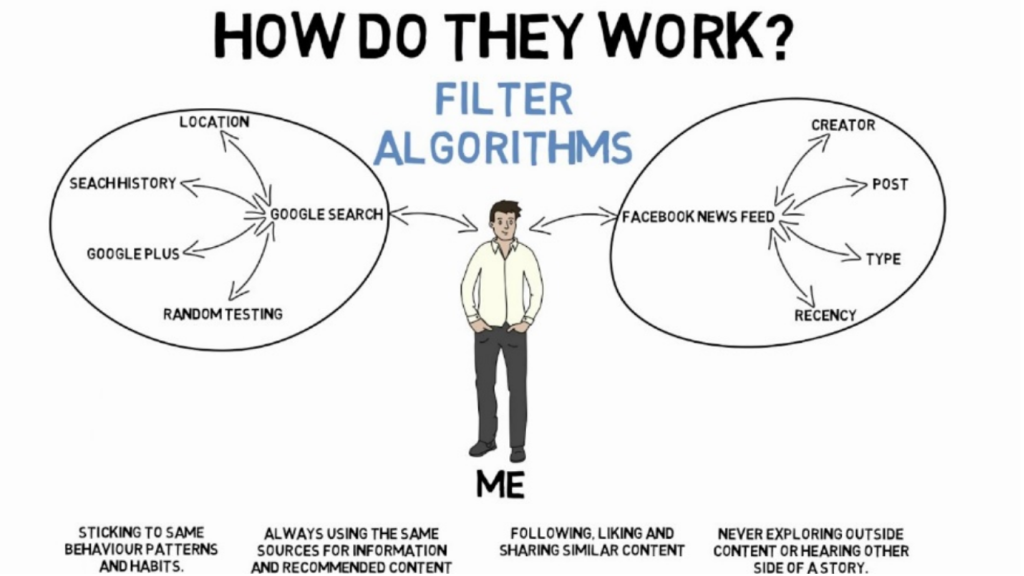
Graph 2: How Filter Bubbles Impact Social Attitudes, licensed under Robert Hardy
(Source: https://www.youtube.com/watch?app=desktop&v=3PR9e5AXgMQ., 2023)
2.2 Perpetuating Stereotypes
A concerning manifestation of search engine bias is the perpetuation of stereotypes. For instance, certain search queries related to gender or race might yield results that enforce negative stereotypes. These results not only misinform users but also contribute to the reinforcement of harmful biases in society.
Consider a study conducted by Safiya Umoja Noble (2021) in her book “Algorithms of Oppression: How Search Engines Reinforce Racism”. Noble highlights how, for years, a search for the term “black girls” on a major search engine yielded results that were overwhelmingly sexualized and derogatory1. This reflects, amplifies, and perpetuates racial and gender biases ingrained in societal structures. Similarly, another alarming incident took place when a Google Photos application mistakenly labeled African Americans as “gorillas” (Graph 3). This glaring error spotlighted how racial biases could inadvertently become a part of seemingly neutral machine-learning systems. Gender biases also find their way into search engine results. For example, as indicated by researchers Sigalow & Fox (2014), for a considerable time, typing “women should” into the Google search bar resulted in autocomplete suggestions like “women should stay at home” or “women should be slaves,” revealing deep-seated gender stereotypes. This finding resonates with that of scholar Hansen (2022), highlighting that while these suggestions are generated based on user search behaviors and popular queries, their very presence on such a widely used platform can unconsciously validate and reinforce these harmful stereotypes. These missteps in search algorithms have tangible consequences. They don’t just misinform or mislead individual users; they effectively propagate and cement age-old biases in a digital age, making them harder to challenge and rectify.
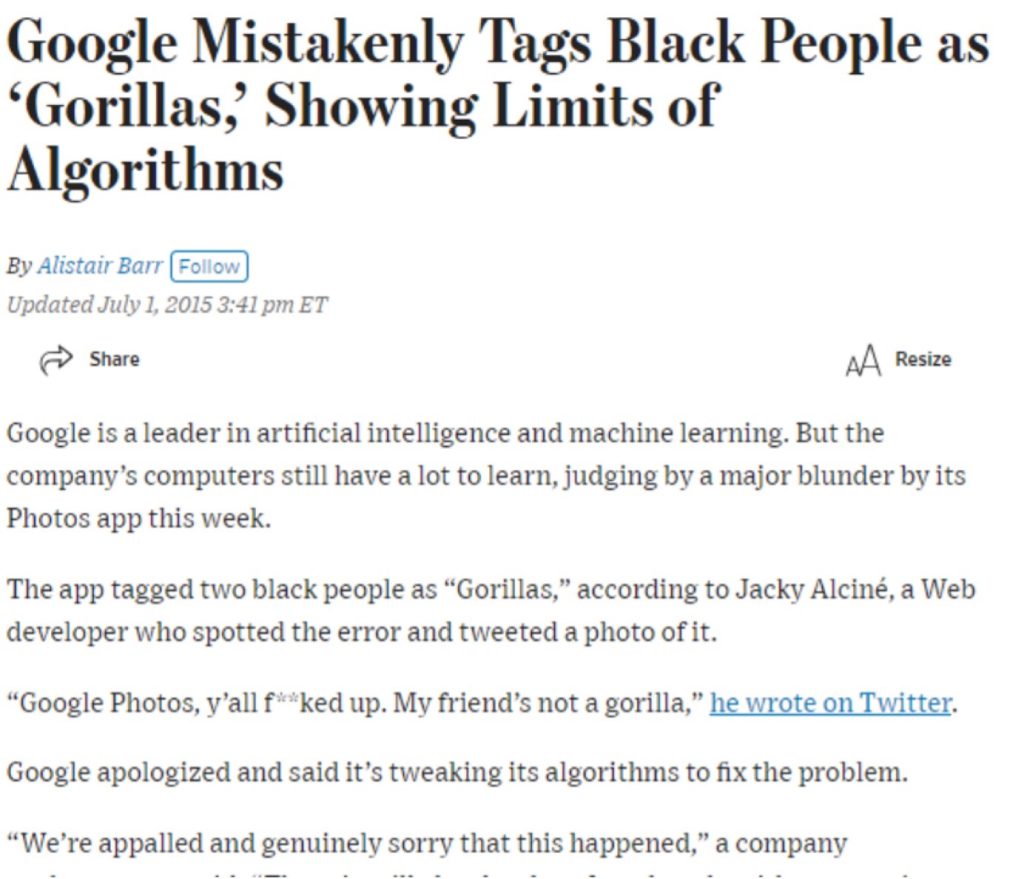
Graph 3: Google Mistakenly Tags Black People as ‘Gorillas,’ Showing Limits of Algorithms, Licensed under WSL
(Source: https://www.wsj.com/articles/BL-DGB-42522 ., 2023)
2.3 Implications for Society
According to the review of “Americans See Search Engines as Biased” posted on platform Statista (Graph 4) by Feldman (2018), search engine biases can deeply impact societal perspectives. They not only skew public opinion and potentially influence election outcomes but also amplify societal divisions through a reinforcing feedback loop of biased results and user interactions. For instance, as indicated in the research “Shifting attention to accuracy can reduce misinformation online” carried out by Pennycook (2021), altering search result rankings could shift the voting preferences of undecided individuals by a significant percentage over 20%. In close elections, such a shift could be decisive. Furthermore, the global spread of misinformation during elections underscores how search engines can prioritize sensational or unverified content, often because of its clickbait nature, thereby further distorting public understanding.
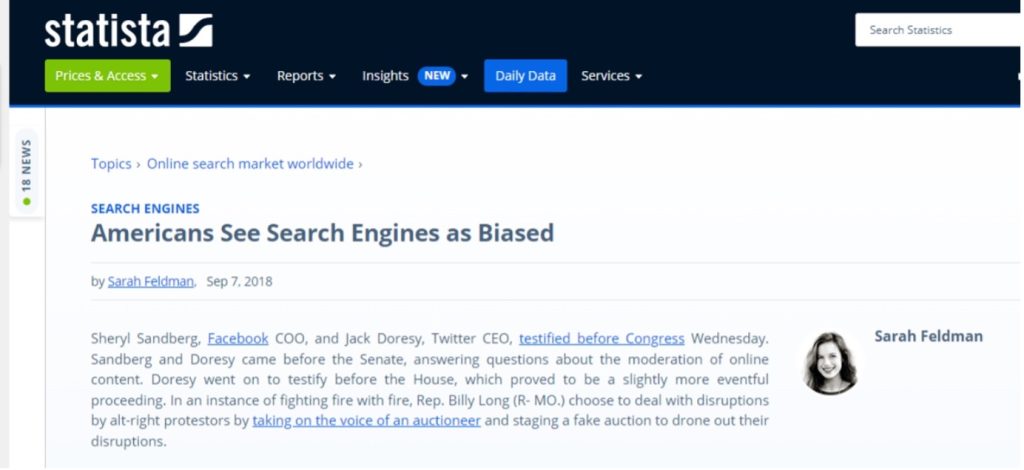
Graph 4: Search Engines as Biased, Licensed under Sarah Feldman.
(Source:https://www.statista.com/chart/15385/americans-see-search-enginges-as-biased/ ., 2023)
3. Counterarguments & Reconciliation
At the intersection of the debate on search engine bias stands a central argument: search engines are merely reflecting societal tendencies and preferences (Bartlett, 2019), not creating, or amplifying them. This viewpoint positions search engines as digital canvases that transparently depict our inherent biases, prejudices, and inclinations. However, as noted by Hicks (2018), those algorithms are crafted to serve user intentions, mining from vast amounts of data to generate seemingly relevant results. However, this does not exonerate them from the responsibility of perpetuating biases. In fact, it may compound the issue.
Scholar Hicks (2019) illustrates his counterarguments with an analogy, where there is a lake that reflects the scenery around it – the trees, the sky, the mountains. However, a search engine is more akin to a mirror in a funhouse. While it does reflect, it can also distort, enlarge, or minimize what it displays, based on intricate calculations that the viewer (user) might not fully perceive. When a user searches for a term and clicks on a particular link, the algorithm registers it as a relevant result for that query. Over time, this result becomes predominant with thousands or millions of similar interactions, pushing other potentially relevant content to obscurity. This mechanism, rather than being a neutral reflection, intensifies specific biases, making them more pronounced and ingrained in the digital sphere. Moreover, by continually presenting skewed results and receiving user engagement in return, search engines develop a reinforcing feedback loop. This isn’t just passive reflection; it’s active reinforcement (Hicks, 2018). Consider the implications for younger generations who might rely on these platforms for education and worldview formation. Over time, as suggested by scholar Bhamore (2019), an algorithmic nudge can shift into a societal shove, pushing entire communities toward a warped sense of ‘normal’.
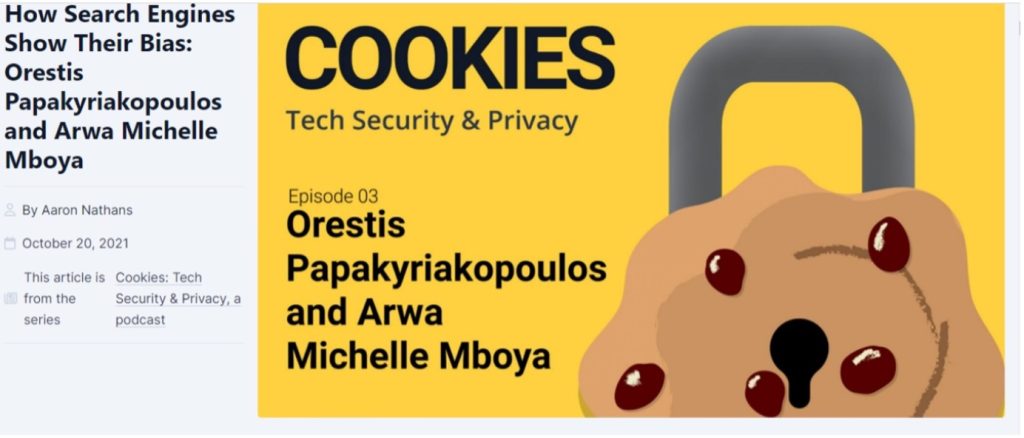
Graph 5: How Search Engines Show Their Bias? Licensed under princeton.edu
(Source: https://engineering.princeton.edu/news/2021/10/20/ ., 2023)
In reconciling these contradicting viewpoints, it becomes evident that while search engines might not be the genesis of societal biases, they are pivotal players in their perpetuations and magnifications. Rather than absolving them based on their foundational intent, it’s critical to continually scrutinize their output and advocate for greater transparency and fairness in their operations. As suggested in a media post titled “How Search Engines Show Their Bias” by Aaron (2021) (Graph 5), while search engines might argue that these outcomes are based on aggregate user data, it’s evident that the algorithm amplifies existing societal prejudices, further entrenching them in the digital realm. These instances highlight a crucial point: search engines, while designed to be neutral, operate in a feedback loop. They present results based on perceived user preferences, which are shaped by societal biases. When users engage with these biased results, the algorithm registers this engagement as validation of the content’s relevance, pushing such content higher up in future searches (Graph 6). This cyclical process not only reinforces but also magnifies existing biases, subtly guiding users toward a skewed version of reality. In essence, while search engines may initiate their journey as reflections of societal perspectives, their inherent design and the feedback loops they create ensure that they play an active role in shaping, reinforcing, and sometimes exacerbating those very biases.
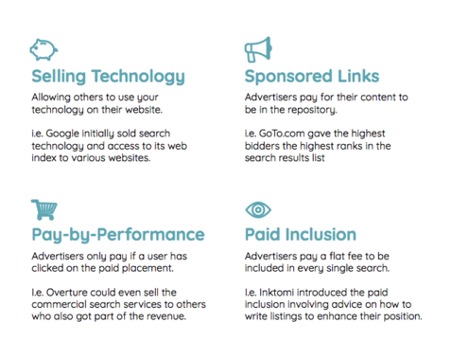
Graph 6: Counterarguments & Reconciliations: The neutrality of search engines, Licensed under statista
(Source:https://www.statista.com/chart/15385/americans-see-search-enginges-as-biased/ ., 2023)
Conclusion
Search engines, while indispensable tools for modern-day information retrieval, are not devoid of biases. Recognizing, addressing, and mitigating these biases is crucial to ensuring that they truly serve as neutral and reliable gatekeepers of information.
References
- Bartlett, J. (2019). Masked by Trust: Bias in Library Discovery. Online Searcher, 43(5), pp.61–62. https://scholarworks.gvsu.edu/cgi/viewcontent.cgi?article=1029&context=library_books
- Bhamore, S. (2019). Decrypting Google’s Search Engine Bias Case: Anti-Trust Enforcement in the Digital Age. Christ University law journal, 8(1), pp.37–60. https://journals.christuniversity.in/index.php/culj/article/view/1905
- Bonart, M., Samokhina, A., Heisenberg, G., & Schaer, P. (2019). An investigation of biases in web search engine query suggestions. Online Information Review, 44(2), 365–381. https://doi.org/10.1108/OIR-11-2018-0341
- Feldman, S. (2018). Americans see search engines as biased. Statista. https://www.statista.com/chart/15385/americans-see-search-enginges-as-biased/
- Fell, E. (2019). Search Engine Society (Digital Media and Society). European Journal of Communication, 34(5), pp.564–566. https://kateto.net/
- Hansen, C. (2022). Dismantling or perpetuating gender stereotypes. – The case of trans rights in the European Court of Human Rights’ jurisprudence. The Age of Human Rights, 18, 143–161. https://doi.org/10.17561/tahrj.v18.7022
- Hicks, M. (2018). Fixing Tech’s Built-In Bias.(Scientists’ Nightstand). American Scientist, 106(5), pp.314–316.
- Nathans, A. (2021, October 20). How search engines show their bias: Orestis Papakyriakopoulos and Arwa Michelle Mboya. Cookies: Tech Security & Privacy. https://engineering.princeton.edu/news/2021/10/20/how-search-engines-show-their-bias-orestis-papakyriakopoulos-and-arwa-michelle-mboya
- Noble, S. U. (2021). Algorithms of oppression: How search engines reinforce racism. NYU Press. https://doi.org/10.1126/science.abm5861
- Pennycook, G., Epstein, Z., Mosleh, M., & et al. (2021). Shifting attention to accuracy can reduce misinformation online. Nature, 592, 590–595. https://doi.org/10.1038/s41586-021-03344-2
- Sigalow, E., & Fox, N. S. (2014). Perpetuating Stereotypes: A Study of Gender, Family, and Religious Life in Jewish Children’s Books. Journal for the Scientific Study of Religion, 53(2), 416–431. https://doi.org/10.1111/jssr.12112
- van Dalen, A. (2023). Algorithmic Gatekeeping for Professional Communicators: Power, Trust, and Legitimacy. Taylor & Francis Group. https://doi.org/10.4324/9781003375258