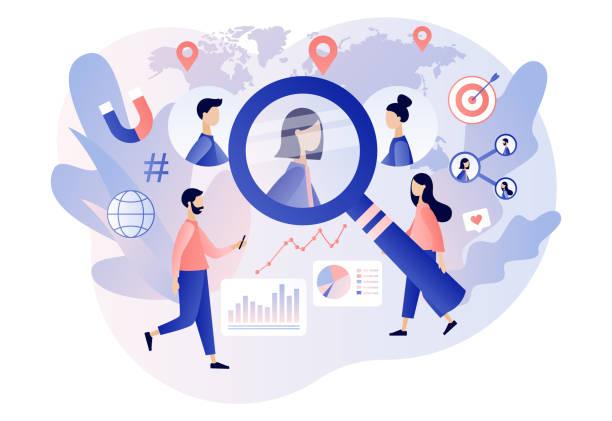
The practice of target marketing has become a prominent force in the digital landscape, which brings forefront the critical issue of ethical concerns stemming from algorithmic bias, a foundational element of target marketing. By investigating real-world examples, it becomes evident how marginalised groups are disproportionately targeted and negatively impact. This underscores the importance of addressing these issues to foster fairness, equality, and transparency in the digital realm.
Defining Target Marketing
Target marketing involves showing specific ads only to a particular audience while concealing them from the general public (Bhootra, 2021). Targeted consumers are usually more receptive compared to the approach of mass marketing, as the messages are precisely crafted to resonate with their specific preferences and needs. Implicit persuasion used in target marketing subtly reinforces existing behaviour patterns (whether those of the individuals themselves or those within the same segmented groups), while also grooming individuals towards desired decisions of marketers, carrying adverse implications (Grier & Kumanyika, 2010). However, this practice relies on data collection and user profiling, a phenomenon that raises important privacy concerns. The sheer volume of personal data gathered in pursuit of precision targeting has sparked debates about the ethical boundaries of data usage, individuals’ right to privacy, and the potential for data breaches or misuse.
In many instances, targeted marketing serves benign purposes, allowing businesses to tailor their marketing efforts for specific customer groups and optimise their advertising strategies. However, targeted marketing also carries the potential for harm. This harm may arise intentionally, as evidenced by Trump’s strategic use of Facebook’s targeted marketing to dissuade African Americans from voting. Alternatively, it can occur inadvertently due to AI algorithms embedded with pre-existing stereotypes.
Algorithmic Biases
Algorithmic bias within machine learning marketing models originates from several factors, including the use of unrepresentative datasets, weak model or algorithm designs, or historical human biases, resulting in unfair outcomes for customers in terms of value creation (Akter et al., 2022). Furthermore, processing unstructured data can generate biased outcomes as it requires deriving generalisable insights from large amounts of unprocessed data, consequently introducing bias into the training dataset as individuals may subjectively assign weights to study variables that align with their personal beliefs, values, and biases (Akter et al., 2022).
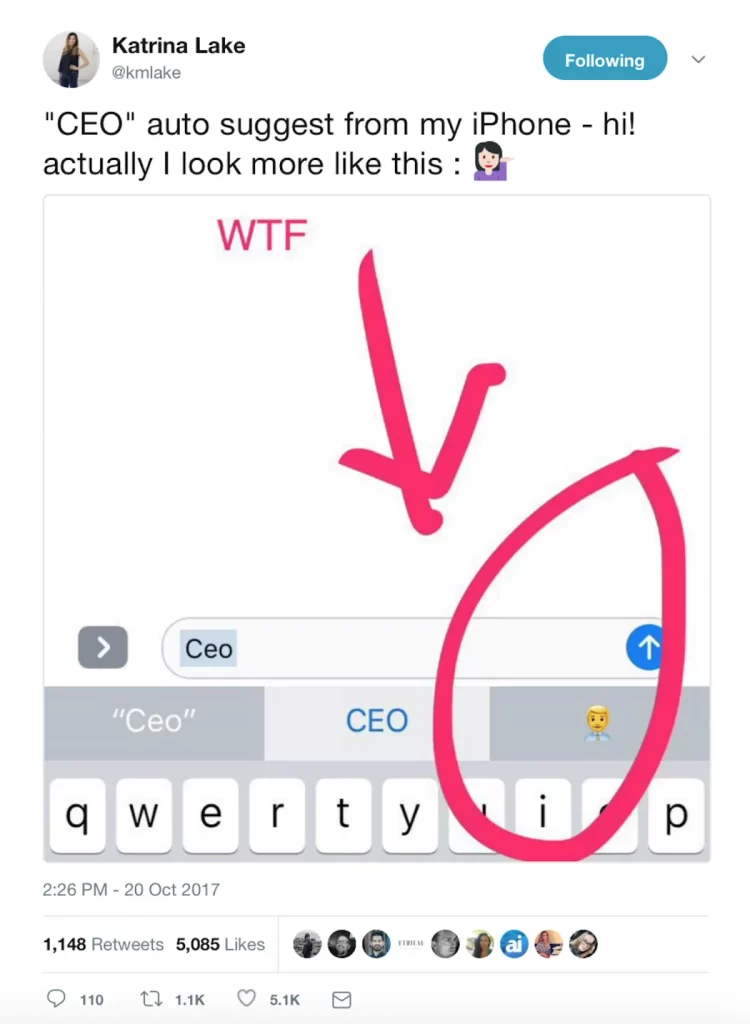
This is exemplified by “word embedding”, a popular data backed model which has picked up on deeply entrenched social biases which leads to services like Google Translate reflecting such biases in their results when translating gender-neutral words, such as “he is an engineer” versus “she is a nurse” (Olsen, 2018). Such examples however are endless, ranging from Uber’s or Lyft’s expensive pricing for areas with large African-American populations, to Optum’s racial bias in medical algorithm for serving patients. (Akter et al., 2022).
Implications of Algorithmic Bias in Target Marketing
Issues with bias surfaces during problem framing when the objective of a machine-learning model is misaligned with the need to avoid discrimination (Bhootra, 2021). Decisions, albeit primarily profit-driven, can yield outcomes that are discriminatory, perpetuating the exclusion of historically marginalised groups (Akter et al., 2022). Furthermore, multicultural marketing and AdTech have started using racial categories to shape their business practices and strategies under the guise of racial diversity and inclusion. However, in the process, these approaches are reinforcing epistemologies and ideologies tied to the cultural politics of racial segregation. Therefore longstanding legacies of racial inequality and reproduced, all the while the rich and powerful accrue more wealth and status (Bui, Chang & McIlwain, 2022). These flaws in the process can manifest as practical issues, such as the distribution of job postings, where positions like preschool teachers and secretaries are shown more to women, while jobs like janitors and taxi drivers are more frequently displayed to people of colour (Bhootra, 2021), or African-American names were more likely to return ads to that person from a service that renders arrest records, as compared to the ad results for white names.
It is worth noting that segmentation and targeting based on factors like gender, race, or social class are not inherently problematic. The ethical concerns arise when these categorisations are used to facilitate unethical practices. Furthermore, the issue extends beyond clear-cut categorisation. Even after Facebook removed racial categories, they developed “affinity” categories based on users’ interests, which preserves and utilises racial stereotypes and assumptions to inform audience segmentation (Bui, Chang & McIlwain, 2022).
While search engines and platforms present themselves as impartial sources of factual and fair information, they are, in reality, heavily tailored and personalised. This misconception can significantly impact individuals’ decisions when searching for jobs, housing, or educational opportunities, potentially leading to negative outcomes.
Exploitation of Vulnerable Populations
It is important to highlight that the problem is far from mere ideological, and that it is disproportionately affecting marginalised groups and exacerbating isolation and division. A stark example from 2015 demonstrates how Google’s targeted advertising facilitated for-profit colleges in preying on isolated individuals with low self-esteem, inadequate support systems, and bleak future prospects. Identifying people with those traits as their target demographic, these colleges primarily admitted a high number of African-American students, pushing them towards pursuing essentially worthless degrees, thus increasing their debt burden and worsening their career prospects (Appel & Taylor, 2015).
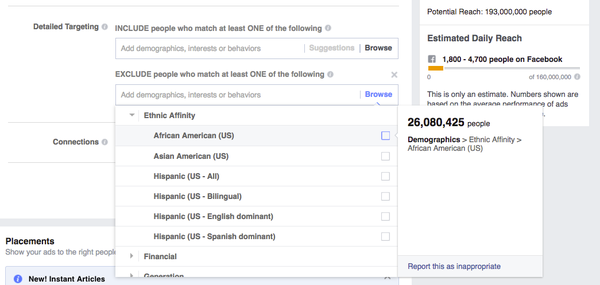
These issues have been ongoing for years, and major platforms like Facebook have consistently found themselves entangled in lawsuits and controversies concerning their discriminatory targeting practices. Despite making public commitments to address these concerns, the problems persist unabated. To illustrate, as far back as 2017, Facebook announced measures to prevent the use of categories for ads related to housing, credit, and jobs. However, Facebook’s marketplace reportedly still allowed housing providers to filter their ads based on factors like family status, disability, and national origin, and their were sued for potentially violating the federal Fair Housing Act (Karlis, 2019). Fast-forwarding to 2021, internal Facebook’s research leaks once again confirmed that they were aware of how vulnerable groups, including children, were preyed upon through the platform’s content recommendations. Thus highlighting the lack of meaningful change from big tech companies in addressing such issues (Bui, Chang & McIlwain, 2022).
Regulations and Oversight
These issues have not gone unnoticed by lawmakers worldwide. In the United States, a multitude of proposed solutions, including the recent passage of the Algorithmic Accountability Act of 2022, exemplify a dedicated effort to foster a culture of accountability and transparency regarding the utilisation of automated systems by companies. This legislation mandates monitoring and reporting any potential negative impacts of AI algorithms. However, it is noteworthy that in Australia, there have been no discernible attempts to introduce new legislation or conduct a comprehensive review of existing legal frameworks to address discrimination arising from algorithmic systems (Sheard, 2022).
On a theoretical front, research on algorithmic bias in machine learning-based marketing models remains largely anecdotal and fragmented, lacking integration into a comprehensive conceptual framework. To make meaningful progress, it is imperative to clearly define the sources and dimensions of bias and to develop ethical frameworks for responsible use of AI (Akter et al., 2022).
Potential solutions span from individual actions to changes in public policy. Operators of algorithms should adopt a proactive approach by developing bias impact statements as part of their processes. Furthermore, existing nondiscrimination and civil rights laws should be modernised to encompass and redress disparate impacts in online contexts (Lee et al., 2019). Nevertheless, it is undeniable that regulating such practices poses formidable challenges, necessitating the development of a clearer framework and approach for standardised auditing and documentation processes. Attempts should extend beyond individual de-biasing efforts into broader meso- and macro-level campaigns aimed at rectifying systemic biases (Bui, Chang & McIlwain, 2022).
Bibliography
Akter, S., Dwivedi, Y. K., Sajib, S., Biswas, K., Bandara, R. J. & Micheal, K. (2022). Algorithmic bias in machine learning-based marketing models. Journal of Business Research, 144(1), 201-216. https://doi.org/10.1016/j.jbusres.2022.01.083
Appel, H. & Taylor, A. (2015). Education with a Debt Sentence: For-Profit Colleges as American Dream Crushers and Factories of Debt. New Labor Forum, 24(1), 31-36. http://www.jstor.org/stable/24718567
Bhootra, G. (2021, August 27). Bias in AI algorithms of Target Advertisements. LinkedIn. https://www.linkedin.com/pulse/bias-ai-algorithms-target-advertisements-ghazal-bhootra/
Bui, M., Chang, H-C. H. & McIlwan, C. (2022, November). Targeted Ads: The Infrastructure for Algorithmic Discrimination. Centre for Critical Race + Digital Studies. https://www.criticalracedigitalstudies.com
Danner, C. (2021, October 27). What Is Being Leaked in the Facebook Papers? A guide to the biggest revelations. Intelligencer. https://nymag.com/intelligencer/2021/10/what-was-leaked-in-the-facebook-papers.html
Grier, S. A. & Kumanyika, S. (2010). Targeted Marketing and Public Health. Annual Review of Public Health, 31(1), 349-369. https://doi.org/10.1146/annurev.publhealth.012809.103607
Karlis, N. (2019, August 21). “Digital redlining”: Facebook’s housing ads seem designed to discriminate. Salon. https://www.salon.com/2019/08/21/digital-redlining-facebooks-housing-ads-seem-designed-to-discriminate/
Lee, N. T, Resnick, P. & Barton, G. (2019, May 22). Algorithmic bias detection and mitigation: Best practices and policies to reduce consumer harms. Brookings. https://www.brookings.edu/articles/algorithmic-bias-detection-and-mitigation-best-practices-and-policies-to-reduce-consumer-harms/
Olsen, P. (2018, February 15). The Algorithm That Helped Google Translate Become Sexist. Forbes. https://www.forbes.com/sites/parmyolson/2018/02/15/the-algorithm-that-helped-google-translate-become-sexist/?sh=17269b157daa
Sheard, N. (2022). Employment Discrimination by Algorithm: Can Anyone Be Held Accountable? UNSW Law Journal, 45(2), 617-648. https://doi.org/10.53637/XTQY4027
White, G. (2017, March 7). When Algorithms Don’t Account for Civil Rights. The Atlantic. https://www.theatlantic.com/business/archive/2017/03/facebook-ad-discrimination/518718/