Engine algorithms are often relied upon to improve decision-making efficiency and objectivity. However, systematic social biases are found in the output of these algorithms. Gender bias in widely used Internet search algorithms reflects the level of gender inequality that exists within society. Algorithmic output gender bias can lead people to think and act in ways that exacerbate social inequality.
WHAT IS ENGINE ALGORITHM AND ITS GENDER BIAS?
Stereotypes and bias are widely distributed in every aspect of social life, and with the advent of Web 2.0 era, this phenomenon has penetrated the virtual cyber-society even more silently. What exactly causes this bias to show up on search engines and how does the inanimate engine produce such conscious bias? It really all comes down to a function called search engine algorithm. A search engine is a collection of formulas that determine the quality and relevance of a particular advert or webpage to a customer query, if you love a particular film on Netflix or a term you searched for on a shopping platform, this will all be part of the algorithmic trap. Search engines are biased towards putting the user experience at the forefront of them, Google is a prime example of a search engine that has succeeded in becoming the most popular search engine on the planet because of its ability to use its algorithms to improve the search process in order to provide the user with the information that they are seeking (Caroline C, 2022).
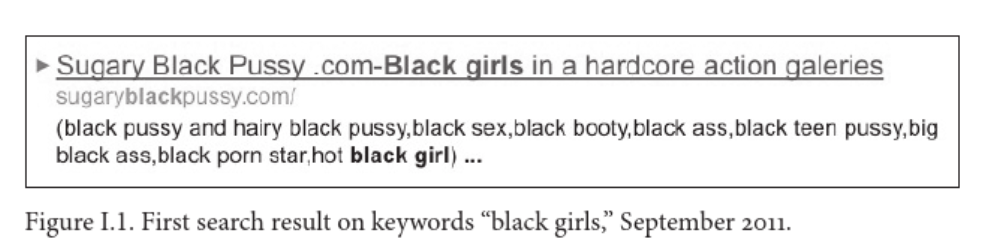
A vivid example of this is the negative messages that come up when searching for colored race or women in Google, as Nobel (2018) mentions in his article that when he typed in the keyword “black girl”, the search results popped up with a number of topics about sexually suggestive nicknames, pornography, or racism. While these results were disabled in a subsequent algorithm update, it’s not hard to imagine that they were corrected because of the huge negative impact they had, it’s disheartening that minorities such as Latina and Asian women are still common victims of these harmful biases now. Considering the immediacy of this example, search results from five years ago may have changed by now, the following images are some of my own Google searches for sensitive terms that may appear biased.
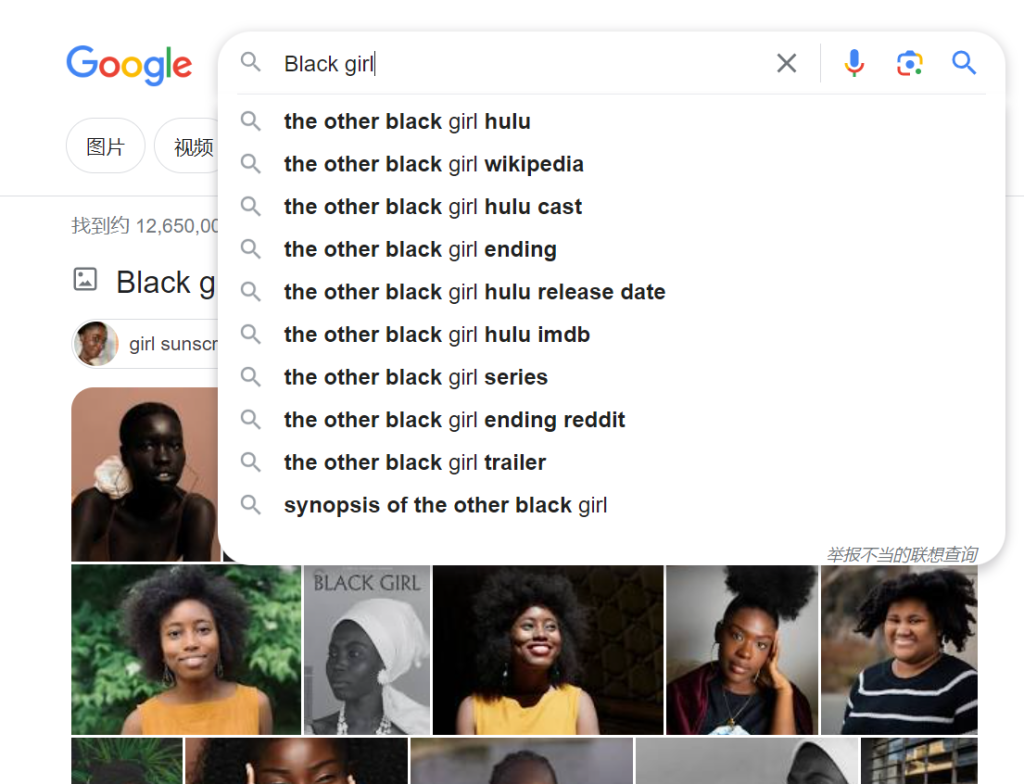
The search proves that Google should have put a lot of effort into this area, and it is now more difficult to visually detect the presence of bias in the relevant keyword associations and the images that appear.
WHAT MAKES GENDER BIAS IN ALGORITHM?
However, the reality is that gender-neutral Internet searches produce results that still male-dominated information output. Psychologists have shown that these sexist search results can exacerbate gender bias and thus influence hiring decisions or have an impact on the users’ own thinking due to data training embedded in social biases (NYU, 2022). The harmful of algorithmic bias can be significant, the retail platform Amazon has 60% of men in its global workforce and 74% of men in managerial positions, the reason for this is that the algorithmic data will be submitted to Amazon to build the selection is mainly from white males, whereas resumes that include the word female have higher requirements and higher selection rates (Nicol L., et al, 2019).
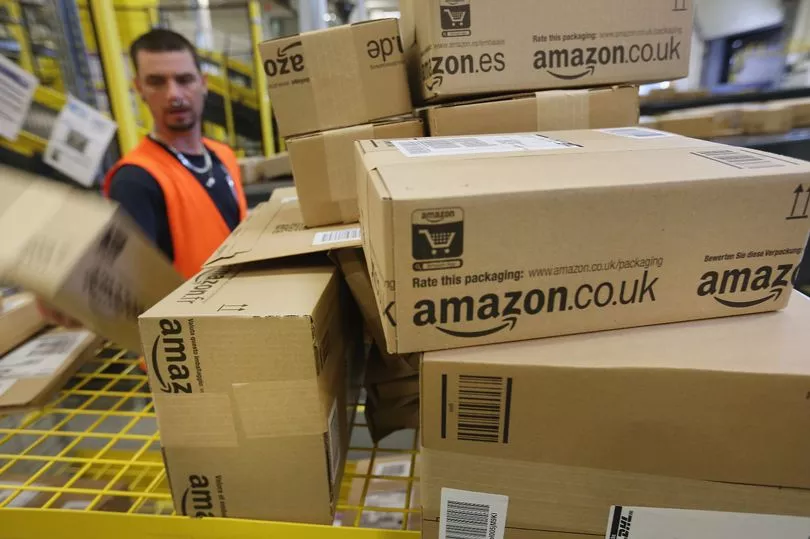
The causes of algorithmic bias are diverse, with the main reasons being historical human bias towards gender, incomplete or unrepresentative data when training algorithms, the fact that algorithms do not have the ability to select for sensitive information that appeals to some users’ search desires. In algorithms, bias in the training data can be devastating, as it represents the possibility that gender bias may have been set in stone from the very beginning of the algorithm’s design. In a cross-country study that ranked countries on gender inequality (GGGI) and then drew on research in psychology: for example, “human” is usually assumed to be male, and word embedding studies trained on large internet corpora found that the word “woman” did not appear alongside “man” which indicate that individuals in society generally exhibit a male default bias (Madalina V et al, 2022). Users utilizing the algorithms to perform tasks will have their databases exercised so that if you are a white male mindset, then the ideal candidate will be of the same racial criteria (LibertiesEU, 2021). There is also a part of the search engines and media that is controlled by the government or stakeholders that use search results to show users what they want to see, so that users are subconsciously accepting those ‘common sense’. Another part of the bias is that Internet companies do not update their databases in time, which leads to the accumulation of various biased information, resulting in default associations in search engines.
HOW TO SOLVE ALGORITHM ON GENDER?
Therefore, to solve algorithm bias, we should start from the following three aspects. Firstly, Internet operating companies and stakeholders should take the initiative to solve the factors leading to bias, they should follow the principle of multicultural and multi-racial multi-orientation when hiring employees, so as to enrich the diversity of the whole system that avoid some tricky groups of employees ignoring their own racial perception bias. Secondly, update the database regularly, manage those search results that tend to cause sensitivity and recheck or block negative information promptly. The government or stakeholders should establish ethical frameworks, such as regulating privacy and data management, writing some terms and conditions, also making users more transparent on the Internet (real-name usage), all of which will limit to a certain extent the desires of some unscrupulous users who want to search and reduce the occurrence of these harmful biases. Certainly, I think algorithms and the biases they create should be viewed critically. There is no doubt that the creation of algorithms does help users make quicker and more rational decisions based on evidence and steps. Algorithms have helped make life easier for people rather than spending more time looking for favorite movie pictures and recipes, while meaning less pollution, less congestion and less waste in the economy (Pernille T, 2017).
CONCLUSION
In general, gender bias caused by engine algorithms does have a negative impact on society and individuals. For example, images and messages that are physically offensive and insulting to women, unfair treatment in recruitment and even some legal disparities in the treatment of colored races and in the sentencing of men and women. It is precisely because algorithm is designed by humans and can be optimized and updated. By diversifying the recruitment from the community, updating the speed of the information data base and the diversity of feeding data, running a regulated ethical template can all go some way to improving the occurrence of such phenomena. Engine algorithms are already moving in the right direction at a rate visible on the road to fighting gender bias and be sure that such biases will be taken more seriously some time soon.
Reference
Caroline, C. (2022). Search Engine Algorithms: What You Need to Know. (2 May). Access from: https://hawksem.com/blog/search-engine-algorithms-what-to-know/
Data Demystified. (2021). Gender Bias in AI and Machine Learning Systems. Access from: https://www.youtube.com/watch?v=Z7-DjqgO2ws
Kirstie M. (2021). Getty Image. Amazon is hiring for seasonal work. Access from: https://www.cambridge-news.co.uk/news/local-news/amazon-jobs-new-seasonal-job-22151453
LibertiesEU. (2021). Algorithmic Bias: Why and How Do Computers Make Unfair Decisions? Access from: https://www.liberties.eu/en/stories/algorithmic-bias-17052021/43528
Madalina V, David M.A. (2022). Propagation of societal gender inequality by internet search algorithms. (July 12). Access from: https://www.pnas.org/doi/10.1073/pnas.2204529119
New York University Education and Social Sciences. (2019). Gender Bias in Search Algorithms Has Effect on Users, New Study Finds. (July 12). Access from: https://www.nyu.edu/about/news-publications/news/2022/july/gender-bias-in-search-algorithms-has-effect-on-users–new-study-.html
Nicol Turner Lee, Paul Resnick, Genie Barton. (2019). Algorithmic bias detection and mitigation: Best practices and policies to reduce consumer harms. (May 22). Access from: https://www.brookings.edu/articles/algorithmic-bias-detection-and-mitigation-best-practices-and-policies-to-reduce-consumer-harms/
Noble, Safiya U. (2018). A society, searching. In Algorithms of Oppression: How search engines reinforce racism. New York: New York University. pp. 15-63. https://ebookcentral-proquest-com.ezproxy.library.sydney.edu.au/lib/usyd/reader.action?docID=4834260&ppg=2
Pernille T. (2017). Experts On The Pros & Cons of Algorithms. (February 18). Access from: https://dataethics.eu/prosconsai/
Be the first to comment on "The Harmful Bias about Gender on Internet Algorithm Engines and Platforms"