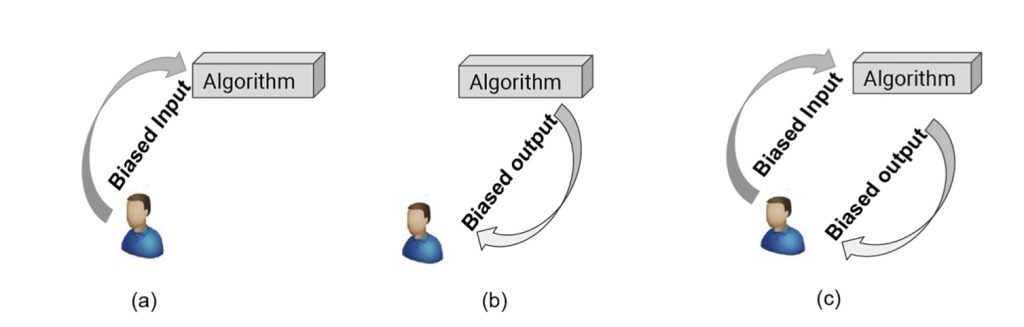
Introduction of Algorithm Bias
In this contemporary digital era, the use of the internet already covers the entire society this day, different digital online platforms provide different functions and services to the public, we use them for social, shopping, for study, for work, and so on. Instead of demonstrating the Internet plays an important role in nowadays society, better to describe this phenomenon that we live in the Internet. Life becomes digital life, it’s another description of the tech culture. However, the algorithm is the key soul for the operation of Internet platforms, it works on the background of the Internet and helps organize, manipulate data to determine the content presented to the users. However, there is a serious phenomenon that exists in the realistic Internet environment, ‘Algorithm Bias’, and the public so relies on and trusts algorithms that Safiya Umoja Noble provides an example in a 2014’s TED Talk that if people want to find a café, they will use google search engine to find which is the most recommended in town. The power of algorithms is an unseen force rising in our daily lives, just like viruses can spread bias on a massive scale without people’s awareness (Joy, 2017). If we separate the words Algorithm and bias, one is just a technique, other one is a word created by society and culture. When they combine, which means there are important interactions between technology and human culture, and how their connection impacts society’s culture and public ideology is the core of this article. Moreover, this article will illustrate how algorithm bias works in this tech culture from 4 different aspects in the following article, which are the facial recognition system, the recommender system, the Medical, and the gender.
Facial Recognition & Algorithm
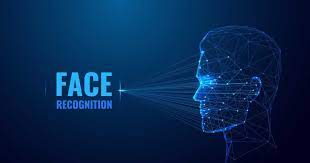
Facial recognition technology as an important identification method was first used to manage criminal photos and crack the criminal case. Now this technology is widely used in various commercial and security systems, recognizing and verifying faces as two main functions in the system. However, the algorithms play a key soul in this technology, and through continually learning the data of different human faces to operate the function in this system. The thing is, the images of different races have differences in recognition accuracy, which affects the function of the recognition system and also causes race bias. Joy Buolamwini’s TED talk in 2017 provided this phenomenon that, she is a black girl and uses the same facial recognition system as her peer, but it just didn’t work on her face when she attended a digital project and an entrepreneurship competition in HK, only if she put on a white mask or using her white classmate’s face, the facial recognition software got the reaction. From Cavazos’s relevant research in 2020, the algorithm itself does not have a bias. The algorithm recognition model always has a higher accuracy for the majority-race face data than the minority, and it doesn’t matter which race is majority or minority. This result suggests that ‘identification accuracy was greater for the race with which the model had greater ‘experience’’(Cavazos et al., 2020). In addition, the algorithm is a product that humans create, algorithm bias originated from human bias, and this deviation begins with the guy who put their stereotype into the algorithm, therefore who code is also important. Programmers support all the technologies we use, but the majority of them are white, male, and young, which leads to bias in the systems and devices they develop (Svensson, 2022). Due to this source, there is a phenomenon that the image of Caucasians is more accurate than the image of black individuals, and over all comparisons, middle-aged White males have the greatest accuracy, but young black females have the lowest accuracy in the VGG-Face algorithm (Cavazos et al., 2020).
Recommender System & Gender Bias
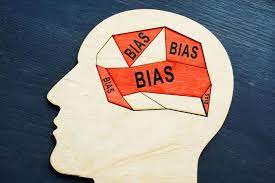
Furthermore, if we research with the most common algorithm that is used in research engines in different website platforms which is called the recommender system, its algorithm bias is represented in the personalization filter. The research article by Wenlong Sun indicates that ‘prominent in personalized user interfaces, can lead to more inequality in estimated relevance and to a limited human ability to discover relevant data’(Sun, et al., 2020). Information retrieval and recommender systems provide services for users to filter the information they are interested in, but the primary sources of bias come from algorithms and humans (Sun, et al., 2020). The model receives almost fixed labels by people’s preference and response to it as a fixed circulate but does not receive the information randomly, that’s the reason why the deviation of the algorithm is being enlarged continually.
In Safiya Umoja Noble’s TED speech in 2014, she showed how the Google research engine represents the image of different races, black girls, and Asia girls always relevant to porn in the search result; When typing Black people in the Google search, negative words showed results as the keyword; Typing ‘Beautiful, white girl’s sexy images showed; All of these are unseen bias created by the algorithm.
Additionally, there are always existing gender biases in the algorithm used in commercial websites. Schroeder’s research reveals ‘how algorithms pick up cultural biases and feed them back into the online environment’(Schroeder, 2021), and women are targeted with more ads for goods, because they traditionally control household expenses (Lambrecht & Tuckrt,2019, p2976). From my observation, when I used me and my boyfriend’s phone to search for dumbbells on Taobao (Chinese shopping website), Obvious algorithm bias occurred.
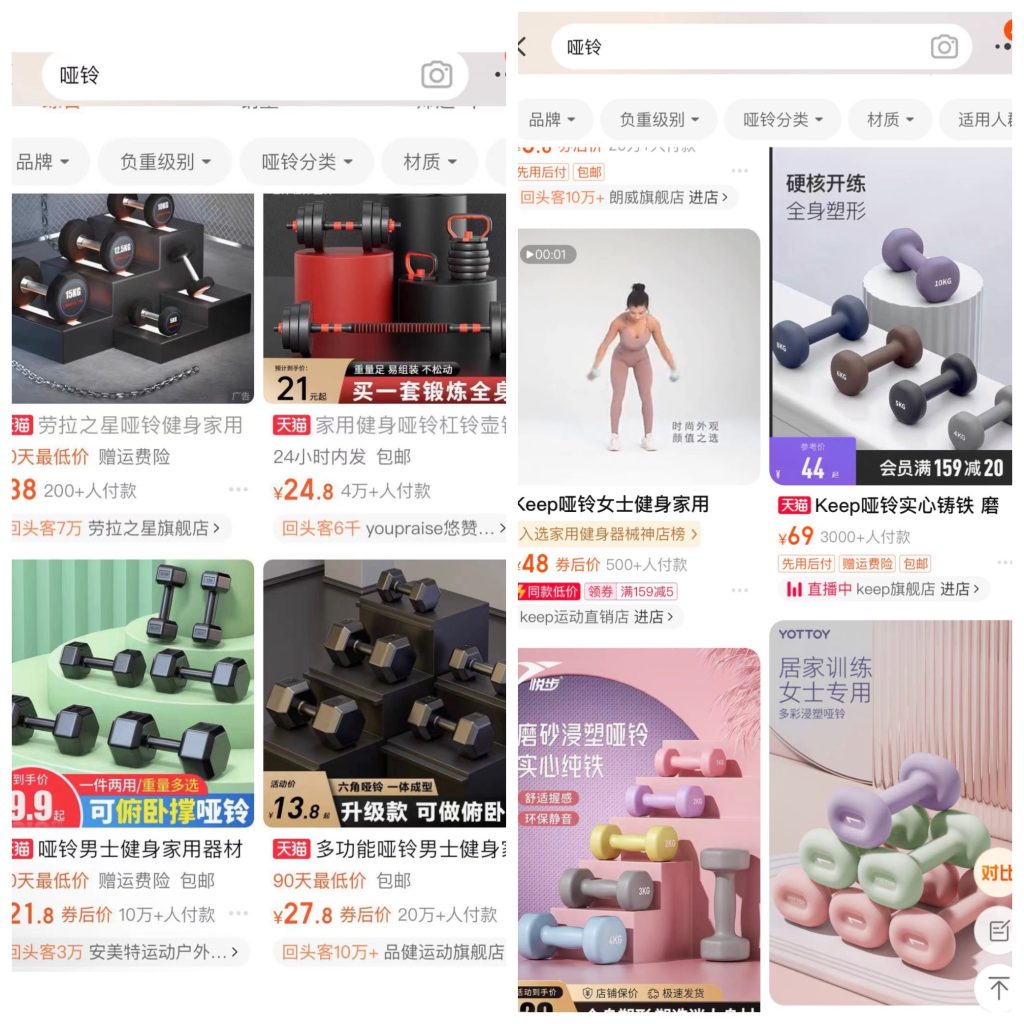
In Figure 3, search for the same product on the same shopping website but the algorithm bias uses stereotypes to classify it into female images and male images, and obviously, the barbells used by women have a richer appearance and also more expensive price than those used by men.
Medical & Algorithm Bias
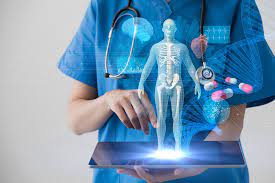
Moreover, algorithm bias is also present in the intelligent medical system. Jain’s relevant article provides an awareness of how algorithm bias impacts patients to experience unfair treatment because of different races and genders. For example, in the algorithm used to estimate kidney function, when the individuals are otherwise similar, the system will determine black person has a healthier kidney than a white person when compared with these two races of patient, which means the white person will be determined as the patient who is more in need of priority treatment and resources (Jain et al., 2023). In addition, the article from Richardson also pointed out algorithm impacts by the human culture bias that cultural belief of “stoic” males causes boys to be given a higher pain score than girls (Richardson, 2022).
Core For Algorithm Bias
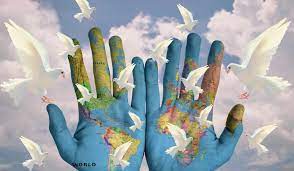
‘Rather than being biological or genetic, race is socially constructed’ (Jain et al., 2023). All in all, this article aims to warn the society and public about how algorithms manipulate people’s ideology and recognition potentially, they occur in the background of society, and quietly plant the seeds of prejudice in people’s minds. The world is diverse, of course, whether stereotyped, racist, or biased we must admit their existence in human culture and society, every individual has their own mindset and choice to decide their cognition and opinion, but there is no doubt that the right of decision making must be based on the known, otherwise there will not exist real freedom. Although, the development of technology has provided unprecedented convenience to human society and changed all modern ways of life. However, in contrast, for instance, algorithms will be the example that when it is no longer controllable, or even the person who created it can no longer control over its self-development, we should and must have a basic understanding of this phenomenon in advance to make some preparations for the mind. For the ordinary public, recognizing this black casework allows us to detect some hints promptly to react in our brains, and also respond to it with the most critical thinking to ideologies that may be intentionally implanted by biased algorithms. Moreover, in this digital age, people can utilize the convenience of social media platforms to quickly complete a large amount of human interaction, and everyone is the sender and the receiver in the meantime in this common shared community, which is called the ritual model (Carey, 2009). Based on this information transmission environment, human commonsense has continually evolved, because the public’s ideology is supported by a billion people’s different perspectives. We share, we argue, so we improve. Therefore, algorithms as a double-edged sword, they can be the most convenient technological tool for humans, but never be the barrier to hindering diverse voices by hundreds of different voices, and a single source of information should never be the truth.
Reference list:
- Svensson, J. (2022). Modern Mathemagics: Values and Biases in Tech Culture. In Systemic Bias (pp. 21–39). https://doi.org/10.4324/9781003173373-2
- Cavazos, J. G., Phillips, P. J., Castillo, C. D., & O’Toole, A. J. (2021). Accuracy Comparison Across Face Recognition Algorithms: Where Are We on Measuring Race Bias? IEEE Transactions on Biometrics, Behavior, and Identity Science, 3(1), 101–111. https://doi.org/10.1109/TBIOM.2020.3027269
- Schroeder, J. E. (2021). Reinscribing gender: social media, algorithms, bias. Journal of Marketing Management, 37(3-4), 376–378. https://doi.org/10.1080/0267257X.2020.1832378
- Jain, A., Brooks, J. R., Alford, C. C., Chang, C. S., Mueller, N. M., Umscheid, C. A., & Bierman, A. S. (2023). Awareness of Racial and Ethnic Bias and Potential Solutions to Address Bias With Use of Health Care Algorithms. JAMA Health Forum, 4(6), E231197–e231197. https://doi.org/10.1001/jamahealthforum.2023.1197
- Sun, W., Nasraoui, O., & Shafto, P. (2020). Evolution and impact of bias in human and machine learning algorithm interaction. PloS One, 15(8), e0235502–e0235502. https://doi.org/10.1371/journal.pone.0235502
- Richardson, A. (2022). Biased data lead to biased algorithms. Canadian Medical Association Journal, 194(9), E341–E341. https://doi.org/10.1503/cmaj.80860
- TEDx Talks. (2014, April 19). How biased are our algorithms? | Safiya Umoja Noble | TEDxUIUC. Www.youtube.com. https://www.youtube.com/watch?v=UXuJ8yQf6dI&t=16s
- TED. (2017). How I’m Fighting Bias in Algorithms | Joy Buolamwini [YouTube Video]. In YouTube. https://www.youtube.com/watch?v=UG_X_7g63rY
- Lambrecht, A., & Tucker, C. (2019). Algorithmic bias? An empirical study of apparent gender-based discrimination in the display of STEM career ads. Management Science, 65(7), 2966–2981.
- Carey, J. W. (2009). A Cultural Approach to Communication. In New Media (p. 199–). SAGE Publications Ltd.
Be the first to comment on "What is Algorithm Bias?"